Open Access
ARTICLE
Image Super-Resolution Reconstruction Based on the DSSTU-Net Model
1 School of Electronic Engineering and Automation, Guilin University of Electronic Technology, Guilin, 541004, China
2 School of Architecture and Transportation Engineering, Guilin University of Electronic Technology, Guilin, 541004, China
3 Key Laboratory of Intelligence Integrated Automation in Guangxi Universities, Guilin, 541004, China
* Corresponding Author: Peng Sun. Email:
(This article belongs to the Special Issue: Computer Vision and Image Processing: Feature Selection, Image Enhancement and Recognition)
Computers, Materials & Continua 2025, 83(1), 1057-1078. https://doi.org/10.32604/cmc.2025.059946
Received 21 October 2024; Accepted 21 January 2025; Issue published 26 March 2025
Abstract
Super-resolution (SR) reconstruction addresses the challenge of enhancing image resolution, which is critical in domains such as medical imaging, remote sensing, and computational photography. High-quality image reconstruction is essential for enhancing visual details and improving the accuracy of subsequent tasks. Traditional methods, including interpolation techniques and basic CNNs, often fail to recover fine textures and detailed structures, particularly in complex or high-frequency regions. In this paper, we present Deep Supervised Swin Transformer U-Net (DSSTU-Net), a novel architecture designed to improve image SR by integrating Residual Swin Transformer Blocks (RSTB) and Deep Supervision (DS) mechanisms into the U-Net framework. DSSTU-Net leverages the Swin Transformer’s multi-scale attention capabilities for robust feature extraction, while DS at various stages of the network ensures better gradient propagation and refined feature learning. The ST block introduces a hierarchical self-attention mechanism, allowing the model to capture both local and global context, which is crucial for high-quality SR tasks. Moreover, DS applied at multiple layers in the decoder enables direct supervision on intermediate feature maps, accelerating convergence and improving performance. The DSSTU-Net architecture was rigorously evaluated on the DIV2K, LSDIR, SET5, and SET14 datasets, demonstrating its superior ability to generate high-quality images. Furthermore, the potential applications of this model extend beyond image enhancement, with promising use cases in medical imaging, satellite imagery, and industrial inspection, where high-quality image reconstruction plays a crucial role in accurate diagnostics and operational efficiency. This work provides a reference method for future research on advanced image restoration techniques.Keywords
Cite This Article
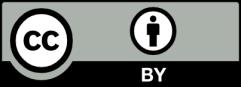
This work is licensed under a Creative Commons Attribution 4.0 International License , which permits unrestricted use, distribution, and reproduction in any medium, provided the original work is properly cited.