Open Access
ARTICLE
Enhancing Adversarial Example Transferability via Regularized Constrained Feature Layer
1 School of Computer Science and Technology, Chongqing University of Posts and Telecommunications, Chongqing, 400065, China
2 Chongqing Key Laboratory of Public Big Data Security Technology, Chongqing, 401420, China
3 School of Cyber Security and Information Law, Chongqing University of Posts and Telecommunications, Chongqing, 400065, China
4 Key Laboratory of Cyberspace Big Data Intelligent Security, Ministry of Education, Chongqing, 400065, China
5 Artificial Intelligence and Big Data College, Chongqing Polytechnic University of Electronic Technology, Chongqing, 401331, China
* Corresponding Author: Long Chen. Email:
Computers, Materials & Continua 2025, 83(1), 157-175. https://doi.org/10.32604/cmc.2025.059863
Received 18 October 2024; Accepted 06 January 2025; Issue published 26 March 2025
Abstract
Transfer-based Adversarial Attacks (TAAs) can deceive a victim model even without prior knowledge. This is achieved by leveraging the property of adversarial examples. That is, when generated from a surrogate model, they retain their features if applied to other models due to their good transferability. However, adversarial examples often exhibit overfitting, as they are tailored to exploit the particular architecture and feature representation of source models. Consequently, when attempting black-box transfer attacks on different target models, their effectiveness is decreased. To solve this problem, this study proposes an approach based on a Regularized Constrained Feature Layer (RCFL). The proposed method first uses regularization constraints to attenuate the initial examples of low-frequency components. Perturbations are then added to a pre-specified layer of the source model using the back-propagation technique, in order to modify the original adversarial examples. Afterward, a regularized loss function is used to enhance the black-box transferability between different target models. The proposed method is finally tested on the ImageNet, CIFAR-100, and Stanford Car datasets with various target models, The obtained results demonstrate that it achieves a significantly higher transfer-based adversarial attack success rate compared with baseline techniques.Keywords
Cite This Article
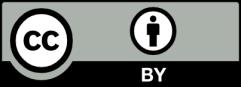
This work is licensed under a Creative Commons Attribution 4.0 International License , which permits unrestricted use, distribution, and reproduction in any medium, provided the original work is properly cited.