Open Access
ARTICLE
Target Detection-Oriented RGCN Inference Enhancement Method
1 School of Internet of Things Engineering, Wuxi University, Wuxi, 214105, China
2 College of Computer Science and Engineering, Changchun University of Technology, Changchun, 130012, China
3 Computer Science and Technology at Guohao Academy, Tongji University, Shanghai, 200092, China
4 China North Vehicle Research Institute, Beijing, 100072, China
* Corresponding Author: Yutong Jiang. Email:
(This article belongs to the Special Issue: Novel Methods for Image Classification, Object Detection, and Segmentation)
Computers, Materials & Continua 2025, 83(1), 1219-1237. https://doi.org/10.32604/cmc.2025.059856
Received 18 October 2024; Accepted 21 January 2025; Issue published 26 March 2025
Abstract
In this paper, a reasoning enhancement method based on RGCN (Relational Graph Convolutional Network) is proposed to improve the detection capability of UAV (Unmanned Aerial Vehicle) on fast-moving military targets in urban battlefield environments. By combining military images with the publicly available VisDrone2019 dataset, a new dataset called VisMilitary was built and multiple YOLO (You Only Look Once) models were tested on it. Due to the low confidence problem caused by fuzzy targets, the performance of traditional YOLO models on real battlefield images decreases significantly. Therefore, we propose an improved RGCN inference model, which improves the performance of the model in complex environments by optimizing the data processing and graph network architecture. Experimental results show that the proposed method achieves an improvement of 0.4% to 1.7% on mAP@0.50, which proves the effectiveness of the model in military target detection. The research of this paper provides a new technical path for UAV target detection in urban battlefield, and provides important enlightenment for the application of deep learning in military field.Keywords
Cite This Article
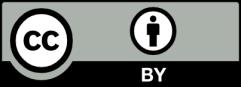
This work is licensed under a Creative Commons Attribution 4.0 International License , which permits unrestricted use, distribution, and reproduction in any medium, provided the original work is properly cited.