Open Access
ARTICLE
A Global-Local Parallel Dual-Branch Deep Learning Model with Attention-Enhanced Feature Fusion for Brain Tumor MRI Classification
School of Computer Science and Engineering, Hunan University of Science and Technology, Xiangtan, 411100, China
* Corresponding Author: Xinlian Zhou. Email:
(This article belongs to the Special Issue: Medical Imaging Based Disease Diagnosis Using AI)
Computers, Materials & Continua 2025, 83(1), 739-760. https://doi.org/10.32604/cmc.2025.059807
Received 17 October 2024; Accepted 02 January 2025; Issue published 26 March 2025
Abstract
Brain tumor classification is crucial for personalized treatment planning. Although deep learning-based Artificial Intelligence (AI) models can automatically analyze tumor images, fine details of small tumor regions may be overlooked during global feature extraction. Therefore, we propose a brain tumor Magnetic Resonance Imaging (MRI) classification model based on a global-local parallel dual-branch structure. The global branch employs ResNet50 with a Multi-Head Self-Attention (MHSA) to capture global contextual information from whole brain images, while the local branch utilizes VGG16 to extract fine-grained features from segmented brain tumor regions. The features from both branches are processed through designed attention-enhanced feature fusion module to filter and integrate important features. Additionally, to address sample imbalance in the dataset, we introduce a category attention block to improve the recognition of minority classes. Experimental results indicate that our method achieved a classification accuracy of and a micro-average Area Under the Curve (AUC) of 0.989 in the classification of three types of brain tumors, surpassing several existing pre-trained Convolutional Neural Network (CNN) models. Additionally, feature interpretability analysis validated the effectiveness of the proposed model. This suggests that the method holds significant potential for brain tumor image classification.Keywords
Cite This Article
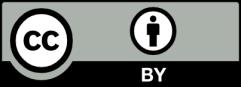
This work is licensed under a Creative Commons Attribution 4.0 International License , which permits unrestricted use, distribution, and reproduction in any medium, provided the original work is properly cited.