Open Access
ARTICLE
An Uncertainty Quantization-Based Method for Anti-UAV Detection in Infrared Images
1 School of Information and Software Engineering, University of Electronic Science and Technology of China, Chengdu, 610054, China
2 State Key Laboratory of Air Traffic Management System, Nanjing, 210000, China
3 National Airspace Management Center, Beijing, 100094, China
* Corresponding Author: Yunbo Rao. Email:
(This article belongs to the Special Issue: Computer Vision and Image Processing: Feature Selection, Image Enhancement and Recognition)
Computers, Materials & Continua 2025, 83(1), 1415-1434. https://doi.org/10.32604/cmc.2025.059797
Received 17 October 2024; Accepted 23 December 2024; Issue published 26 March 2025
Abstract
Infrared unmanned aerial vehicle (UAV) target detection presents significant challenges due to the interplay between small targets and complex backgrounds. Traditional methods, while effective in controlled environments, often fail in scenarios involving long-range targets, high noise levels, or intricate backgrounds, highlighting the need for more robust approaches. To address these challenges, we propose a novel three-stage UAV segmentation framework that leverages uncertainty quantification to enhance target saliency. This framework incorporates a Bayesian convolutional neural network capable of generating both segmentation maps and probabilistic uncertainty maps. By utilizing uncertainty predictions, our method refines segmentation outcomes, achieving superior detection accuracy. Notably, this marks the first application of uncertainty modeling within the context of infrared UAV target detection. Experimental evaluations on three publicly available infrared UAV datasets demonstrate the effectiveness of the proposed framework. The results reveal significant improvements in both detection precision and robustness when compared to state-of-the-art deep learning models. Our approach also extends the capabilities of encoder-decoder convolutional neural networks by introducing uncertainty modeling, enabling the network to better handle the challenges posed by small targets and complex environmental conditions. By bridging the gap between theoretical uncertainty modeling and practical detection tasks, our work offers a new perspective on enhancing model interpretability and performance. The codes of this work are available openly at (acceessed on 11 November 2024).Keywords
Cite This Article
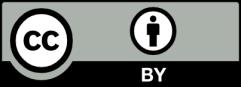
This work is licensed under a Creative Commons Attribution 4.0 International License , which permits unrestricted use, distribution, and reproduction in any medium, provided the original work is properly cited.