Open Access
ARTICLE
Institution Attribute Mining Technology for Access Control Based on Hybrid Capsule Network
Henan Province Key Laboratory of Information Security, Information Engineering University, Zhengzhou, 450000, China
* Corresponding Author: Xuehui Du. Email:
(This article belongs to the Special Issue: Applications of Artificial Intelligence for Information Security)
Computers, Materials & Continua 2025, 83(1), 1471-1489. https://doi.org/10.32604/cmc.2025.059784
Received 16 October 2024; Accepted 14 January 2025; Issue published 26 March 2025
Abstract
Security attributes are the premise and foundation for implementing Attribute-Based Access Control (ABAC) mechanisms. However, when dealing with massive volumes of unstructured text big data resources, the current attribute management methods based on manual extraction face several issues, such as high costs for attribute extraction, long processing times, unstable accuracy, and poor scalability. To address these problems, this paper proposes an attribute mining technology for access control institutions based on hybrid capsule networks. This technology leverages transfer learning ideas, utilizing Bidirectional Encoder Representations from Transformers (BERT) pre-trained language models to achieve vectorization of unstructured text data resources. Furthermore, we have designed a novel end-to-end parallel hybrid network structure, where the parallel networks handle global and local information features of the text that they excel at, respectively. By employing techniques such as attention mechanisms, capsule networks, and dynamic routing, effective mining of security attributes for access control resources has been achieved. Finally, we evaluated the performance level of the proposed attribute mining method for access control institutions through experiments on the medical referral text resource dataset. The experimental results show that, compared with baseline algorithms, our method adopts a parallel network structure that can better balance global and local feature information, resulting in improved overall performance. Specifically, it achieves a comprehensive performance enhancement of 2.06% to 8.18% in the F1 score metric. Therefore, this technology can effectively provide attribute support for access control of unstructured text big data resources.Keywords
Cite This Article
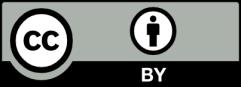
This work is licensed under a Creative Commons Attribution 4.0 International License , which permits unrestricted use, distribution, and reproduction in any medium, provided the original work is properly cited.