Open Access
ARTICLE
UniTrans: Unified Parameter-Efficient Transfer Learning and Multimodal Alignment for Large Multimodal Foundation Model
1 Chengdu Institute of Computer Application, Chinese Academy of Sciences, Chengdu, 610213, China
2 School of Computer Science and Technology, University of Chinese Academy of Sciences, Beijing, 101499, China
* Corresponding Author: Cheng Peng. Email:
Computers, Materials & Continua 2025, 83(1), 219-238. https://doi.org/10.32604/cmc.2025.059745
Received 16 October 2024; Accepted 08 January 2025; Issue published 26 March 2025
Abstract
With the advancements in parameter-efficient transfer learning techniques, it has become feasible to leverage large pre-trained language models for downstream tasks under low-cost and low-resource conditions. However, applying this technique to multimodal knowledge transfer introduces a significant challenge: ensuring alignment across modalities while minimizing the number of additional parameters required for downstream task adaptation. This paper introduces UniTrans, a framework aimed at facilitating efficient knowledge transfer across multiple modalities. UniTrans leverages Vector-based Cross-modal Random Matrix Adaptation to enable fine-tuning with minimal parameter overhead. To further enhance modality alignment, we introduce two key components: the Multimodal Consistency Alignment Module and the Query-Augmentation Side Network, specifically optimized for scenarios with extremely limited trainable parameters. Extensive evaluations on various cross-modal downstream tasks demonstrate that our approach surpasses state-of-the-art methods while using just 5% of their trainable parameters. Additionally, it achieves superior performance compared to fully fine-tuned models on certain benchmarks.Keywords
Cite This Article
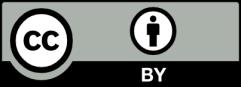
This work is licensed under a Creative Commons Attribution 4.0 International License , which permits unrestricted use, distribution, and reproduction in any medium, provided the original work is properly cited.