Open Access
ARTICLE
Enhanced Detection of APT Vector Lateral Movement in Organizational Networks Using Lightweight Machine Learning
1 Research and Innovation Center, Rabdan Academy, Abudhabi, P.O. Box 114646, United Arab Emirates
2 UQ Cyber Research Centre, The University of Queensland, Brisbane, QLD 4027, Australia
3 Department of Computer Science, Bingham University, Abuja, 961105, Nigeria
4 School of Computing, Robert Gordon University, Aberdeen, AB10 7QB, UK
5 College of Technological Innovation, Zayed University, Dubai, P.O. Box 144534, United Arab Emirates
* Corresponding Author: Mathew Nicho. Email:
Computers, Materials & Continua 2025, 83(1), 281-308. https://doi.org/10.32604/cmc.2025.059597
Received 12 October 2024; Accepted 28 November 2024; Issue published 26 March 2025
Abstract
The successful penetration of government, corporate, and organizational IT systems by state and non-state actors deploying APT vectors continues at an alarming pace. Advanced Persistent Threat (APT) attacks continue to pose significant challenges for organizations despite technological advancements in artificial intelligence (AI)-based defense mechanisms. While AI has enhanced organizational capabilities for deterrence, detection, and mitigation of APTs, the global escalation in reported incidents, particularly those successfully penetrating critical government infrastructure has heightened concerns among information technology (IT) security administrators and decision-makers. Literature review has identified the stealthy lateral movement (LM) of malware within the initially infected local area network (LAN) as a significant concern. However, current literature has yet to propose a viable approach for resource-efficient, real-time detection of APT malware lateral movement within the initially compromised LAN following perimeter breach. Researchers have suggested the nature of the dataset, optimal feature selection, and the choice of machine learning (ML) techniques as critical factors for detection. Hence, the objective of the research described here was to successfully demonstrate a simplified lightweight ML method for detecting the LM of APT vectors. While the nearest detection rate achieved in the LM domain within LAN was 99.89%, as reported in relevant studies, our approach surpassed it, with a detection rate of 99.95% for the modified random forest (RF) classifier for dataset 1. Additionally, our approach achieved a perfect 100% detection rate for the decision tree (DT) and RF classifiers with dataset 2, a milestone not previously reached in studies within this domain involving two distinct datasets. Using the ML life cycle methodology, we deployed K-nearest neighbor (KNN), support vector machine (SVM), DT, and RF on three relevant datasets to detect the LM of APTs at the affected LAN prior to data exfiltration/destruction. Feature engineering presented four critical APT LM intrusion detection (ID) indicators (features) across the three datasets, namely, the source port number, the destination port number, the packets, and the bytes. This study demonstrates the effectiveness of lightweight ML classifiers in detecting APT lateral movement after network perimeter breach. It contributes to the field by proposing a non-intrusive network detection method capable of identifying APT malware before data exfiltration, thus providing an additional layer of organizational defense.Keywords
Cite This Article
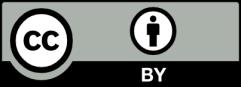
This work is licensed under a Creative Commons Attribution 4.0 International License , which permits unrestricted use, distribution, and reproduction in any medium, provided the original work is properly cited.