Open Access
ARTICLE
xCViT: Improved Vision Transformer Network with Fusion of CNN and Xception for Skin Disease Recognition with Explainable AI
1 Department of Electrical Engineering, Wah Engineering College, University of Wah, Wah Cantt, 47040, Pakistan
2 Department of Computer Science, HITEC University, Taxila, 47080, Pakistan
3 Department of Applied Informatics, Vytautas Magnus University, Kaunas, 44309, Lithuania
* Corresponding Author: Robertas Damaševičius. Email:
Computers, Materials & Continua 2025, 83(1), 1367-1398. https://doi.org/10.32604/cmc.2025.059301
Received 03 October 2024; Accepted 19 February 2025; Issue published 26 March 2025
Abstract
Skin cancer is the most prevalent cancer globally, primarily due to extensive exposure to Ultraviolet (UV) radiation. Early identification of skin cancer enhances the likelihood of effective treatment, as delays may lead to severe tumor advancement. This study proposes a novel hybrid deep learning strategy to address the complex issue of skin cancer diagnosis, with an architecture that integrates a Vision Transformer, a bespoke convolutional neural network (CNN), and an Xception module. They were evaluated using two benchmark datasets, HAM10000 and Skin Cancer ISIC. On the HAM10000, the model achieves a precision of 95.46%, an accuracy of 96.74%, a recall of 96.27%, specificity of 96.00% and an F1-Score of 95.86%. It obtains an accuracy of 93.19%, a precision of 93.25%, a recall of 92.80%, a specificity of 92.89% and an F1-Score of 93.19% on the Skin Cancer ISIC dataset. The findings demonstrate that the model that was proposed is robust and trustworthy when it comes to the classification of skin lesions. In addition, the utilization of Explainable AI techniques, such as Grad-CAM visualizations, assists in highlighting the most significant lesion areas that have an impact on the decisions that are made by the model.Keywords
Cite This Article
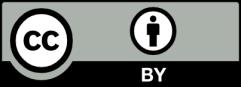
This work is licensed under a Creative Commons Attribution 4.0 International License , which permits unrestricted use, distribution, and reproduction in any medium, provided the original work is properly cited.