Open Access
ARTICLE
GD-YOLO: A Network with Gather and Distribution Mechanism for Infrared Image Detection of Electrical Equipment
1 Key Laboratory of Modern Power System Simulation and Control & Renewable Energy Technology, Ministry of Education, Northeast Electric Power University, Jilin, 132012, China
2 School of Electrical Engineering, Northeast Electric Power University, Jilin, 132012, China
* Corresponding Author: Junpeng Wu. Email:
Computers, Materials & Continua 2025, 83(1), 897-915. https://doi.org/10.32604/cmc.2025.058714
Received 19 September 2024; Accepted 16 January 2025; Issue published 26 March 2025
Abstract
As technologies related to power equipment fault diagnosis and infrared temperature measurement continue to advance, the classification and identification of infrared temperature measurement images have become crucial in effective intelligent fault diagnosis of various electrical equipment. In response to the increasing demand for sufficient feature fusion in current real-time detection and low detection accuracy in existing networks for Substation fault diagnosis, we introduce an innovative method known as Gather and Distribution Mechanism-You Only Look Once (GD-YOLO). Firstly, a partial convolution group is designed based on different convolution kernels. We combine the partial convolution group with deep convolution to propose a new Grouped Channel-wise Spatial Convolution (GCSConv) that compensates for the information loss caused by spatial channel convolution. Secondly, the Gather and Distribute Mechanism, which addresses the fusion problem of different dimensional features, has been implemented by aligning and sharing information through aggregation and distribution mechanisms. Thirdly, considering the limitations in current bounding box regression and the imbalance between complex and simple samples, Maximum Possible Distance Intersection over Union (MPDIoU) and Adaptive SlideLoss is incorporated into the loss function, allowing samples near the Intersection over Union (IoU) to receive more attention through the dynamic variation of the mean Intersection over Union. The GD-YOLO algorithm can surpass YOLOv5, YOLOv7, and YOLOv8 in infrared image detection for electrical equipment, achieving a mean Average Precision (mAP) of 88.9%, with accuracy improvements of 3.7%, 4.3%, and 3.1%, respectively. Additionally, the model delivers a frame rate of 48 FPS, which aligns with the precision and velocity criteria necessary for the detection of infrared images in power equipment.Keywords
Cite This Article
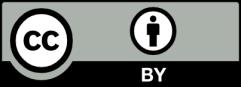
This work is licensed under a Creative Commons Attribution 4.0 International License , which permits unrestricted use, distribution, and reproduction in any medium, provided the original work is properly cited.