Open Access
ARTICLE
Image Copy-Move Forgery Detection and Localization Method Based on Sequence-to-Sequence Transformer Structure
School of Computer Science, Guangdong Polytechnic Normal University, Guangzhou, 510630, China
* Corresponding Author: Peng Liang. Email:
(This article belongs to the Special Issue: Multimedia Security in Deep Learning)
Computers, Materials & Continua 2025, 82(3), 5221-5238. https://doi.org/10.32604/cmc.2025.055739
Received 29 April 2024; Accepted 23 December 2024; Issue published 06 March 2025
Abstract
In recent years, the detection of image copy-move forgery (CMFD) has become a critical challenge in verifying the authenticity of digital images, particularly as image manipulation techniques evolve rapidly. While deep convolutional neural networks (DCNNs) have been widely employed for CMFD tasks, they are often hindered by a notable limitation: the progressive reduction in spatial resolution during the encoding process, which leads to the loss of critical image details. These details are essential for the accurate detection and localization of image copy-move forgery. To overcome the limitations of existing methods, this paper proposes a Transformer-based approach for CMFD and localization as an alternative to conventional DCNN-based techniques. The proposed method employs a Transformer structure as an encoder to process images in a sequence-to-sequence manner, substituting the feature correlation calculations of previous methods with self-attention computations. This allows the model to capture long-range dependencies and contextual nuances within the image, preserving finer details that are typically lost in DCNN-based approaches. Moreover, an appropriate decoder is utilized to ensure precise reconstruction of image features, thereby enhancing both the detection accuracy and localization precision. Experimental results demonstrate that the proposed model achieves superior performance on benchmark datasets, such as USCISI, for image copy-move forgery detection. These results show the potential of Transformer architectures in advancing the field of image forgery detection and offer promising directions for future research.Keywords
Cite This Article
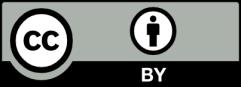
This work is licensed under a Creative Commons Attribution 4.0 International License , which permits unrestricted use, distribution, and reproduction in any medium, provided the original work is properly cited.