Open Access
ARTICLE
MACLSTM: A Weather Attributes Enabled Recurrent Approach to Appliance-Level Energy Consumption Forecasting
1 NARI-TECH Nanjing Control System Co., Ltd., Nanjing, 211106, China
2 School of Software, Nanjing University of Information Science & Technology, Nanjing, 210044, China
3 Jiangsu Province Engineering Research Center of Advanced Computing and Intelligent Services, Nanjing, 210044, China
* Corresponding Author: Ruoxin Li. Email:
Computers, Materials & Continua 2025, 82(2), 2969-2984. https://doi.org/10.32604/cmc.2025.060230
Received 27 October 2024; Accepted 29 December 2024; Issue published 17 February 2025
Abstract
Studies to enhance the management of electrical energy have gained considerable momentum in recent years. The question of how much energy will be needed in households is a pressing issue as it allows the management plan of the available resources at the power grids and consumer levels. A non-intrusive inference process can be adopted to predict the amount of energy required by appliances. In this study, an inference process of appliance consumption based on temporal and environmental factors used as a soft sensor is proposed. First, a study of the correlation between the electrical and environmental variables is presented. Then, a resampling process is applied to the initial data set to generate three other subsets of data. All the subsets were evaluated to deduce the adequate granularity for the prediction of the energy demand. Then, a cloud-assisted deep neural network model is designed to forecast short-term energy consumption in a residential area while preserving user privacy. The solution is applied to the consumption data of four appliances elected from a set of real household power data. The experiment results show that the proposed framework is effective for estimating consumption with convincing accuracy.Keywords
Cite This Article
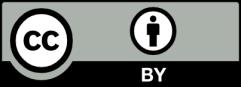
This work is licensed under a Creative Commons Attribution 4.0 International License , which permits unrestricted use, distribution, and reproduction in any medium, provided the original work is properly cited.