Open Access
REVIEW
An Iterative PRISMA Review of GAN Models for Image Processing, Medical Diagnosis, and Network Security
1 Department of Computer Science and Engineering, Prasad V Potluri Siddhartha Institute of Technology, Vijayawada, 520007, India
2 Department of Computer Science and Engineering, Koneru Lakshmaiah Education Foundation, Vaddeswaram, 522302, India
3 Department of Electronics and Communication Engineering, Sreenidhi Institute of Science and Technology, Hyderabad, 501301, India
4 Amrita School of Computing, Amrita Vishwa Vidyapeetham, Amaravati, 522503, India
5 Department of Teleinformatics Engineering, Federal University of Ceará, Fortaleza, 60455-970, Brazil
6 Inti International University, Nilai, 71800, Malaysia
* Corresponding Author: Uddagiri Sirisha. Email:
Computers, Materials & Continua 2025, 82(2), 1757-1810. https://doi.org/10.32604/cmc.2024.059715
Received 15 October 2024; Accepted 12 December 2024; Issue published 17 February 2025
Abstract
The growing spectrum of Generative Adversarial Network (GAN) applications in medical imaging, cyber security, data augmentation, and the field of remote sensing tasks necessitate a sharp spike in the criticality of review of Generative Adversarial Networks. Earlier reviews that targeted reviewing certain architecture of the GAN or emphasizing a specific application-oriented area have done so in a narrow spirit and lacked the systematic comparative analysis of the models’ performance metrics. Numerous reviews do not apply standardized frameworks, showing gaps in the efficiency evaluation of GANs, training stability, and suitability for specific tasks. In this work, a systemic review of GAN models using the PRISMA framework is developed in detail to fill the gap by structurally evaluating GAN architectures. A wide variety of GAN models have been discussed in this review, starting from the basic Conditional GAN, Wasserstein GAN, and Deep Convolutional GAN, and have gone down to many specialized models, such as EVAGAN, FCGAN, and SIF-GAN, for different applications across various domains like fault diagnosis, network security, medical imaging, and image segmentation. The PRISMA methodology systematically filters relevant studies by inclusion and exclusion criteria to ensure transparency and replicability in the review process. Hence, all models are assessed relative to specific performance metrics such as accuracy, stability, and computational efficiency. There are multiple benefits to using the PRISMA approach in this setup. Not only does this help in finding optimal models suitable for various applications, but it also provides an explicit framework for comparing GAN performance. In addition to this, diverse types of GAN are included to ensure a comprehensive view of the state-of-the-art techniques. This work is essential not only in terms of its result but also because it guides the direction of future research by pinpointing which types of applications require some GAN architectures, works to improve specific task model selection, and points out areas for further research on the development and application of GANs.Keywords
Supplementary Material
Supplementary Material FileCite This Article
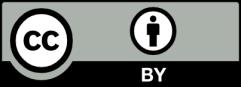
This work is licensed under a Creative Commons Attribution 4.0 International License , which permits unrestricted use, distribution, and reproduction in any medium, provided the original work is properly cited.