Open Access
ARTICLE
Integrating Image Processing Technology and Deep Learning to Identify Crops in UAV Orthoimages
1 Department of Civil Engineering, Republic of China Military Academy, Kaohsiung, 830, Taiwan
2 Department of Marine Science, Republic of China Naval Academy, Kaohsiung, 813, Taiwan
* Corresponding Author: Ching-Lung Fan. Email:
Computers, Materials & Continua 2025, 82(2), 1925-1945. https://doi.org/10.32604/cmc.2025.059245
Received 01 October 2024; Accepted 07 January 2025; Issue published 17 February 2025
Abstract
This study aims to enhance automated crop detection using high-resolution Unmanned Aerial Vehicle (UAV) imagery by integrating the Visible Atmospherically Resistant Index (VARI) with deep learning models. The primary challenge addressed is the detection of bananas interplanted with betel nuts, a scenario where traditional image processing techniques struggle due to color similarities and canopy overlap. The research explores the effectiveness of three deep learning models—Single Shot MultiBox Detector (SSD), You Only Look Once version 3 (YOLOv3), and Faster Region-Based Convolutional Neural Network (Faster RCNN)—using Red, Green, Blue (RGB) and VARI images for banana detection. Results show that VARI significantly improves detection accuracy, with YOLOv3 achieving the best performance, achieving a precision of 73.77%, recall of 100%, and reduced training time by 95 seconds. Additionally, the average Intersection over Union (IoU) increased by 4%–25% across models with VARI-enhanced images. This study confirms that incorporating VARI improves the performance of deep learning models, offering a promising solution for precise crop detection in complex agricultural environments.Keywords
Cite This Article
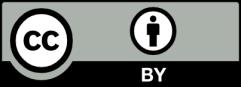
This work is licensed under a Creative Commons Attribution 4.0 International License , which permits unrestricted use, distribution, and reproduction in any medium, provided the original work is properly cited.