Open Access
REVIEW
Machine Learning-Based Routing Protocol in Flying Ad Hoc Networks: A Review
1 Department of Computer Science and Engineering (CSE), Lovely Professional University, Phagwara, 144411, India
2 Department for Program Engineering, Algebra University , Gradiscanska 24, Zagreb, 10000, Croatia
3 Department of Computer Science, College of Computing, Khon Kaen University, Khon Kaen, 40002, Thailand
* Corresponding Author: Arfat Ahmad Khan. Email:
Computers, Materials & Continua 2025, 82(2), 1615-1643. https://doi.org/10.32604/cmc.2025.059043
Received 26 September 2024; Accepted 08 January 2025; Issue published 17 February 2025
Abstract
“Flying Ad Hoc Networks (FANETs)”, which use “Unmanned Aerial Vehicles (UAVs)”, are developing as a critical mechanism for numerous applications, such as military operations and civilian services. The dynamic nature of FANETs, with high mobility, quick node migration, and frequent topology changes, presents substantial hurdles for routing protocol development. Over the preceding few years, researchers have found that machine learning gives productive solutions in routing while preserving the nature of FANET, which is topology change and high mobility. This paper reviews current research on routing protocols and Machine Learning (ML) approaches applied to FANETs, emphasizing developments between 2021 and 2023. The research uses the PRISMA approach to sift through the literature, filtering results from the SCOPUS database to find 82 relevant publications. The research study uses machine learning-based routing algorithms to beat the issues of high mobility, dynamic topologies, and intermittent connection in FANETs. When compared with conventional routing, it gives an energy-efficient and fast decision-making solution in a real-time environment, with greater fault tolerance capabilities. These protocols aim to increase routing efficiency, flexibility, and network stability using ML’s predictive and adaptive capabilities. This comprehensive review seeks to integrate existing information, offer novel integration approaches, and recommend future research topics for improving routing efficiency and flexibility in FANETs. Moreover, the study highlights emerging trends in ML integration, discusses challenges faced during the review, and discusses overcoming these hurdles in future research.Keywords
Supplementary Material
Supplementary Material FileCite This Article
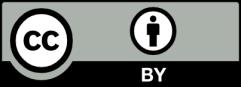
This work is licensed under a Creative Commons Attribution 4.0 International License , which permits unrestricted use, distribution, and reproduction in any medium, provided the original work is properly cited.