Open Access
ARTICLE
Machine Learning-Based Detection and Selective Mitigation of Denial-of-Service Attacks in Wireless Sensor Networks
Department of Future Convergence Technology Engineering, Sungshin Women’s University, Seoul, 02844, Republic of Korea
* Corresponding Author: Il-Gu Lee. Email:
#Co-first authors
(This article belongs to the Special Issue: From Nodes to Knowledge: Harnessing Wireless Sensor Networks)
Computers, Materials & Continua 2025, 82(2), 2475-2494. https://doi.org/10.32604/cmc.2025.058963
Received 25 September 2024; Accepted 13 December 2024; Issue published 17 February 2025
Abstract
As the density of wireless networks increases globally, the vulnerability of overlapped dense wireless communications to interference by hidden nodes and denial-of-service (DoS) attacks is becoming more apparent. There exists a gap in research on the detection and response to attacks on Medium Access Control (MAC) mechanisms themselves, which would lead to service outages between nodes. Classifying exploitation and deceptive jamming attacks on control mechanisms is particularly challengingdue to their resemblance to normal heavy communication patterns. Accordingly, this paper proposes a machine learning-based selective attack mitigation model that detects DoS attacks on wireless networks by monitoring packet log data. Based on the type of detected attack, it implements effective corresponding mitigation techniques to restore performance to nodes whose availability has been compromised. Experimental results reveal that the accuracy of the proposed model is 14% higher than that of a baseline anomaly detection model. Further, the appropriate mitigation techniques selected by the proposed system based on the attack type improve the average throughput by more than 440% compared to the case without a response.Keywords
Cite This Article
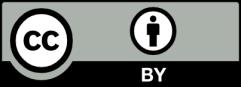
This work is licensed under a Creative Commons Attribution 4.0 International License , which permits unrestricted use, distribution, and reproduction in any medium, provided the original work is properly cited.