Open Access
ARTICLE
External Knowledge-Enhanced Cross-Attention Fusion Model for Tobacco Sentiment Analysis
1 Division of Information Center, Tobacco Sichuan Industry Co., Ltd., Chengdu, 610017, China
2 Computer Network Information Center, Chinese Academy of Sciences, Beijing, 100190, China
* Corresponding Authors: Qing Chen. Email: ; Jun Li. Email:
Computers, Materials & Continua 2025, 82(2), 3381-3397. https://doi.org/10.32604/cmc.2024.058950
Received 24 September 2024; Accepted 03 December 2024; Issue published 17 February 2025
Abstract
In the age of information explosion and artificial intelligence, sentiment analysis tailored for the tobacco industry has emerged as a pivotal avenue for cigarette manufacturers to enhance their tobacco products. Existing solutions have primarily focused on intrinsic features within consumer reviews and achieved significant progress through deep feature extraction models. However, they still face these two key limitations: (1) neglecting the influence of fundamental tobacco information on analyzing the sentiment inclination of consumer reviews, resulting in a lack of consistent sentiment assessment criteria across thousands of tobacco brands; (2) overlooking the syntactic dependencies between Chinese word phrases and the underlying impact of sentiment scores between word phrases on sentiment inclination determination. To tackle these challenges, we propose the External Knowledge-enhanced Cross-Attention Fusion model, CITSA. Specifically, in the Cross Infusion Layer, we fuse consumer comment information and tobacco fundamental information through interactive attention mechanisms. In the Textual Attention Enhancement Layer, we introduce an emotion-oriented syntactic dependency graph and incorporate sentiment-syntactic relationships into consumer comments through a graph convolution network module. Subsequently, the Textual Attention Layer is introduced to combine these two feature representations. Additionally, we compile a Chinese-oriented tobacco sentiment analysis dataset, comprising 55,096 consumer reviews and 2074 tobacco fundamental information entries. Experimental results on our self-constructed datasets consistently demonstrate that our proposed model outperforms state-of-the-art methods in terms of accuracy, precision, recall, and F1-score.Keywords
Cite This Article
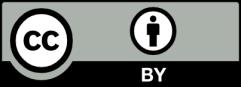
This work is licensed under a Creative Commons Attribution 4.0 International License , which permits unrestricted use, distribution, and reproduction in any medium, provided the original work is properly cited.