Open Access
ARTICLE
MG-SLAM: RGB-D SLAM Based on Semantic Segmentation for Dynamic Environment in the Internet of Vehicles
1 School of Mechanical Engineering, Jiangsu University of Technology, Changzhou, 213001, China
2 School of Automobile and Traffic Engineering, Jiangsu University of Technology, Changzhou, 213001, China
* Corresponding Author: Kai Zhu. Email:
(This article belongs to the Special Issue: Advanced Communication and Networking Technologies for Internet of Things and Internet of Vehicles)
Computers, Materials & Continua 2025, 82(2), 2353-2372. https://doi.org/10.32604/cmc.2024.058944
Received 24 September 2024; Accepted 15 November 2024; Issue published 17 February 2025
Abstract
The Internet of Vehicles (IoV) has become an important direction in the field of intelligent transportation, in which vehicle positioning is a crucial part. SLAM (Simultaneous Localization and Mapping) technology plays a crucial role in vehicle localization and navigation. Traditional Simultaneous Localization and Mapping (SLAM) systems are designed for use in static environments, and they can result in poor performance in terms of accuracy and robustness when used in dynamic environments where objects are in constant movement. To address this issue, a new real-time visual SLAM system called MG-SLAM has been developed. Based on ORB-SLAM2, MG-SLAM incorporates a dynamic target detection process that enables the detection of both known and unknown moving objects. In this process, a separate semantic segmentation thread is required to segment dynamic target instances, and the Mask R-CNN algorithm is applied on the Graphics Processing Unit (GPU) to accelerate segmentation. To reduce computational cost, only key frames are segmented to identify known dynamic objects. Additionally, a multi-view geometry method is adopted to detect unknown moving objects. The results demonstrate that MG-SLAM achieves higher precision, with an improvement from 0.2730 m to 0.0135 m in precision. Moreover, the processing time required by MG-SLAM is significantly reduced compared to other dynamic scene SLAM algorithms, which illustrates its efficacy in locating objects in dynamic scenes.Keywords
Cite This Article
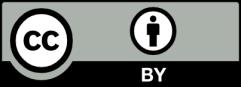
This work is licensed under a Creative Commons Attribution 4.0 International License , which permits unrestricted use, distribution, and reproduction in any medium, provided the original work is properly cited.