Open Access
ARTICLE
Multisource Data Fusion Using MLP for Human Activity Recognition
1 Department of Computer Science and Information Technology, Faculty of Science, Naresuan University, Phitsanulok, 65000, Thailand
2 Center of Excellence for Innovation and Technology for Detection and Advanced Materials (ITDAM), Faculty of Science, Naresuan University, Phitsanulok, 65000, Thailand
3 Center of Excellence in Nonlinear Analysis and Optimizations, Faculty of Science, Naresuan University, Phitsanulok, 65000, Thailand
* Corresponding Author: Kreangsak Tamee. Email:
Computers, Materials & Continua 2025, 82(2), 2109-2136. https://doi.org/10.32604/cmc.2025.058906
Received 24 September 2024; Accepted 02 January 2025; Issue published 17 February 2025
Abstract
This research investigates the application of multisource data fusion using a Multi-Layer Perceptron (MLP) for Human Activity Recognition (HAR). The study integrates four distinct open-source datasets—WISDM, DaLiAc, MotionSense, and PAMAP2—to develop a generalized MLP model for classifying six human activities. Performance analysis of the fused model for each dataset reveals accuracy rates of 95.83 for WISDM, 97 for DaLiAc, 94.65 for MotionSense, and 98.54 for PAMAP2. A comparative evaluation was conducted between the fused MLP model and the individual dataset models, with the latter tested on separate validation sets. The results indicate that the MLP model, trained on the fused dataset, exhibits superior performance relative to the models trained on individual datasets. This finding suggests that multisource data fusion significantly enhances the generalization and accuracy of HAR systems. The improved performance underscores the potential of integrating diverse data sources to create more robust and comprehensive models for activity recognition.Keywords
Cite This Article
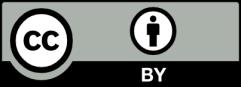
This work is licensed under a Creative Commons Attribution 4.0 International License , which permits unrestricted use, distribution, and reproduction in any medium, provided the original work is properly cited.