Open Access
ARTICLE
Exploratory Research on Defense against Natural Adversarial Examples in Image Classification
The State Key Laboratory of Pulsed Power Laser Technology, National University of Defense Technology, Hefei, 230037, China
* Corresponding Author: Bin Zhu. Email:
Computers, Materials & Continua 2025, 82(2), 1947-1968. https://doi.org/10.32604/cmc.2024.057866
Received 29 August 2024; Accepted 13 November 2024; Issue published 17 February 2025
Abstract
The emergence of adversarial examples has revealed the inadequacies in the robustness of image classification models based on Convolutional Neural Networks (CNNs). Particularly in recent years, the discovery of natural adversarial examples has posed significant challenges, as traditional defense methods against adversarial attacks have proven to be largely ineffective against these natural adversarial examples. This paper explores defenses against these natural adversarial examples from three perspectives: adversarial examples, model architecture, and dataset. First, it employs Class Activation Mapping (CAM) to visualize how models classify natural adversarial examples, identifying several typical attack patterns. Next, various common CNN models are analyzed to evaluate their susceptibility to these attacks, revealing that different architectures exhibit varying defensive capabilities. The study finds that as the depth of a network increases, its defenses against natural adversarial examples strengthen. Lastly, Finally, the impact of dataset class distribution on the defense capability of models is examined, focusing on two aspects: the number of classes in the training set and the number of predicted classes. This study investigates how these factors influence the model’s ability to defend against natural adversarial examples. Results indicate that reducing the number of training classes enhances the model’s defense against natural adversarial examples. Additionally, under a fixed number of training classes, some CNN models show an optimal range of predicted classes for achieving the best defense performance against these adversarial examples.Keywords
Cite This Article
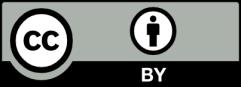
This work is licensed under a Creative Commons Attribution 4.0 International License , which permits unrestricted use, distribution, and reproduction in any medium, provided the original work is properly cited.