Open Access
ARTICLE
Evolutionary Particle Swarm Optimization Algorithm Based on Collective Prediction for Deployment of Base Stations
1 School of Computer Science and Technology, Zhejiang Normal University, Jinhua, 321004, China
2 School of Future Technologies, Jiangxi Institute of Applied Science and Technology, Nanchang, 330000, China
3 School of Computing and Artificial Intelligence, Southwestern University of Finance and Economics, Chengdu, 611130, China
* Corresponding Author: Taiyong Li. Email:
(This article belongs to the Special Issue: Particle Swarm Optimization: Advances and Applications)
Computers, Materials & Continua 2025, 82(1), 345-369. https://doi.org/10.32604/cmc.2024.060335
Received 29 October 2024; Accepted 05 December 2024; Issue published 03 January 2025
Abstract
The wireless signals emitted by base stations serve as a vital link connecting people in today’s society and have been occupying an increasingly important role in real life. The development of the Internet of Things (IoT) relies on the support of base stations, which provide a solid foundation for achieving a more intelligent way of living. In a specific area, achieving higher signal coverage with fewer base stations has become an urgent problem. Therefore, this article focuses on the effective coverage area of base station signals and proposes a novel Evolutionary Particle Swarm Optimization (EPSO) algorithm based on collective prediction, referred to herein as ECPPSO. Introducing a new strategy called neighbor-based evolution prediction (NEP) addresses the issue of premature convergence often encountered by PSO. ECPPSO also employs a strengthening evolution (SE) strategy to enhance the algorithm’s global search capability and efficiency, ensuring enhanced robustness and a faster convergence speed when solving complex optimization problems. To better adapt to the actual communication needs of base stations, this article conducts simulation experiments by changing the number of base stations. The experimental results demonstrate that under the condition of 50 or more base stations, ECPPSO consistently achieves the best coverage rate exceeding 95%, peaking at 99.4400% when the number of base stations reaches 80. These results validate the optimization capability of the ECPPSO algorithm, proving its feasibility and effectiveness. Further ablative experiments and comparisons with other algorithms highlight the advantages of ECPPSO.Keywords
Cite This Article
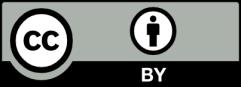
This work is licensed under a Creative Commons Attribution 4.0 International License , which permits unrestricted use, distribution, and reproduction in any medium, provided the original work is properly cited.