Open Access
ARTICLE
Hourglass-GCN for 3D Human Pose Estimation Using Skeleton Structure and View Correlation
Faculty of Robot Science and Engineering, Northeastern University, Shenyang, 110169, China
* Corresponding Author: Chengdong Wu. Email:
Computers, Materials & Continua 2025, 82(1), 173-191. https://doi.org/10.32604/cmc.2024.059284
Received 02 October 2024; Accepted 19 November 2024; Issue published 03 January 2025
Abstract
Previous multi-view 3D human pose estimation methods neither correlate different human joints in each view nor model learnable correlations between the same joints in different views explicitly, meaning that skeleton structure information is not utilized and multi-view pose information is not completely fused. Moreover, existing graph convolutional operations do not consider the specificity of different joints and different views of pose information when processing skeleton graphs, making the correlation weights between nodes in the graph and their neighborhood nodes shared. Existing Graph Convolutional Networks (GCNs) cannot extract global and deep-level skeleton structure information and view correlations efficiently. To solve these problems, pre-estimated multi-view 2D poses are designed as a multi-view skeleton graph to fuse skeleton priors and view correlations explicitly to process occlusion problem, with the skeleton-edge and symmetry-edge representing the structure correlations between adjacent joints in each view of skeleton graph and the view-edge representing the view correlations between the same joints in different views. To make graph convolution operation mine elaborate and sufficient skeleton structure information and view correlations, different correlation weights are assigned to different categories of neighborhood nodes and further assigned to each node in the graph. Based on the graph convolution operation proposed above, a Residual Graph Convolution (RGC) module is designed as the basic module to be combined with the simplified Hourglass architecture to construct the Hourglass-GCN as our 3D pose estimation network. Hourglass-GCN with a symmetrical and concise architecture processes three scales of multi-view skeleton graphs to extract local-to-global scale and shallow-to-deep level skeleton features efficiently. Experimental results on common large 3D pose dataset Human3.6M and MPI-INF-3DHP show that Hourglass-GCN outperforms some excellent methods in 3D pose estimation accuracy.Keywords
Cite This Article
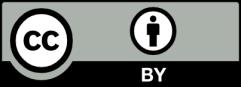
This work is licensed under a Creative Commons Attribution 4.0 International License , which permits unrestricted use, distribution, and reproduction in any medium, provided the original work is properly cited.