Open Access
ARTICLE
Industrial Control Anomaly Detection Based on Distributed Linear Deep Learning
1 School of Computer Science and Information Security, Guilin University of Electronic Technology, Guilin, 541004, China
2 School of Electronic Engineering and Automation, Guilin University of Electronic Technology, Guilin, 541004, China
3 Guangxi Engineering Research Center of Industrial Internet Security and Blockchain, Guilin University of Electronic Technology, Guilin, 541004, China
4 Institute of Cyberspace Technology, HKCT Institute for Higher Education, Hong Kong, 999077, China
5 School of Mathematics & Computing Science, Guilin University of Electronic Technology, Guilin, 541004, China
* Corresponding Author: Yong Ding. Email:
(This article belongs to the Special Issue: Security and Privacy for Blockchain-empowered Internet of Things)
Computers, Materials & Continua 2025, 82(1), 1129-1150. https://doi.org/10.32604/cmc.2024.059143
Received 28 September 2024; Accepted 28 October 2024; Issue published 03 January 2025
Abstract
As more and more devices in Cyber-Physical Systems (CPS) are connected to the Internet, physical components such as programmable logic controller (PLC), sensors, and actuators are facing greater risks of network attacks, and fast and accurate attack detection techniques are crucial. The key problem in distinguishing between normal and abnormal sequences is to model sequential changes in a large and diverse field of time series. To address this issue, we propose an anomaly detection method based on distributed deep learning. Our method uses a bilateral filtering algorithm for sequential sequences to remove noise in the time series, which can maintain the edge of discrete features. We use a distributed linear deep learning model to establish a sequential prediction model and adjust the threshold for anomaly detection based on the prediction error of the validation set. Our method can not only detect abnormal attacks but also locate the sensors that cause anomalies. We conducted experiments on the Secure Water Treatment (SWAT) and Water Distribution (WADI) public datasets. The experimental results show that our method is superior to the baseline method in identifying the types of attacks and detecting efficiency.Keywords
Cite This Article
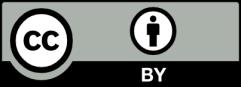
This work is licensed under a Creative Commons Attribution 4.0 International License , which permits unrestricted use, distribution, and reproduction in any medium, provided the original work is properly cited.