Open Access
ARTICLE
A Robust Security Detection Strategy for Next Generation IoT Networks
1 Technology Higher School Essaouira, Cadi Ayyad University, Essaouira, 44000, Morocco
2 IMIA Laboratory, MSIA Team, Faculty of Sciences and Techniques, Moulay Ismail University of Meknes, Errachidia, 50050, Morocco
3 CRIL-CNRS, Artois University, Lens, 62300, France
4 Department of Computer Science and Information Systems, College of Applied Sciences, AlMaarefa University, Diriyah, Riyadh, 13713, Saudi Arabia
5 Department of Computer Science, College of Computer, Qassim University, Buraydah, 52571, Saudi Arabia
* Corresponding Author: Mourade Azrour. Email:
(This article belongs to the Special Issue: Security and Privacy in IoT and Smart City: Current Challenges and Future Directions)
Computers, Materials & Continua 2025, 82(1), 443-466. https://doi.org/10.32604/cmc.2024.059047
Received 27 September 2024; Accepted 03 December 2024; Issue published 03 January 2025
Abstract
Internet of Things (IoT) refers to the infrastructures that connect smart devices to the Internet, operating autonomously. This connectivity makes it possible to harvest vast quantities of data, creating new opportunities for the emergence of unprecedented knowledge. To ensure IoT securit, various approaches have been implemented, such as authentication, encoding, as well as devices to guarantee data integrity and availability. Among these approaches, Intrusion Detection Systems (IDS) is an actual security solution, whose performance can be enhanced by integrating various algorithms, including Machine Learning (ML) and Deep Learning (DL), enabling proactive and accurate detection of threats. This study proposes to optimize the performance of network IDS using an ensemble learning method based on a voting classification algorithm. By combining the strengths of three powerful algorithms, Random Forest (RF), K-Nearest Neighbors (KNN), and Support Vector Machine (SVM) to detect both normal behavior and different categories of attack. Our analysis focuses primarily on the NSL-KDD dataset, while also integrating the recent Edge-IIoT dataset, tailored to industrial IoT environments. Experimental results show significant enhancements on the Edge-IIoT and NSL-KDD datasets, reaching accuracy levels between 72% to 99%, with precision between 87% and 99%, while recall values and F1-scores are also between 72% and 99%, for both normal and attack detection. Despite the promising results of this study, it suffers from certain limitations, notably the use of specific datasets and the lack of evaluations in a variety of environments. Future work could include applying this model to various datasets and evaluating more advanced ensemble strategies, with the aim of further enhancing the effectiveness of IDS.Keywords
Cite This Article
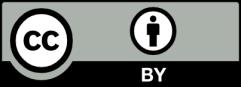
This work is licensed under a Creative Commons Attribution 4.0 International License , which permits unrestricted use, distribution, and reproduction in any medium, provided the original work is properly cited.