Open Access
ARTICLE
Federated Learning’s Role in Next-Gen TV Ad Optimization
Department of Economic Informatics and Cybernetics, Bucharest University of Economic Studies, Bucharest, 010374, Romania
* Corresponding Author: Simona-Vasilica Oprea. Email:
Computers, Materials & Continua 2025, 82(1), 675-712. https://doi.org/10.32604/cmc.2024.058656
Received 17 September 2024; Accepted 08 November 2024; Issue published 03 January 2025
Abstract
In the rapidly evolving landscape of television advertising, optimizing ad schedules to maximize viewer engagement and revenue has become significant. Traditional methods often operate in silos, limiting the potential insights gained from broader data analysis due to concerns over privacy and data sharing. This article introduces a novel approach that leverages Federated Learning (FL) to enhance TV ad schedule optimization, combining the strengths of local optimization techniques with the power of global Machine Learning (ML) models to uncover actionable insights without compromising data privacy. It combines linear programming for initial ads scheduling optimization with ML—specifically, a K-Nearest Neighbors (KNN) model—for predicting ad spot positions. Taking into account the diversity and the difficulty of the ad-scheduling problem, we propose a prescriptive-predictive approach in which first the position of the ads is optimized (using Google’s OR-Tools CP-SAT) and then the scheduled position of all ads will be the result of the optimization problem. Second, this output becomes the target of a predictive task that predicts the position of new entries based on their characteristics ensuring the implementation of the scheduling at large scale (using KNN, Light Gradient Boosting Machine and Random Forest). Furthermore, we explore the integration of FL to enhance predictive accuracy and strategic insight across different broadcasting networks while preserving data privacy. The FL approach resulted in 8750 ads being precisely matched to their optimal category placements, showcasing an alignment with the intended diversity objectives. Additionally, there was a minimal deviation observed, with 1133 ads positioned within a one-category variance from their ideal placement in the original dataset.Keywords
Cite This Article
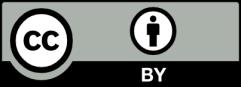
This work is licensed under a Creative Commons Attribution 4.0 International License , which permits unrestricted use, distribution, and reproduction in any medium, provided the original work is properly cited.