Open Access
ARTICLE
A Cross Attention Transformer-Mixed Feedback Video Recommendation Algorithm Based on DIEN
1 College of Software Engineering, Zhengzhou University of Light Industry, Zhengzhou, 450000, China
2 Faculty of Information Engineering, Xuchang Vocational Technical College, Xuchang, 461000, China
3 College of Computer Science and Technology, Zhengzhou University of Light Industry, Zhengzhou, 450000, China
4 College of Electronics and Information, Zhengzhou University of Light Industry, Zhengzhou, 450000, China
* Corresponding Author: Jianwei Zhang. Email:
Computers, Materials & Continua 2025, 82(1), 977-996. https://doi.org/10.32604/cmc.2024.058438
Received 12 September 2024; Accepted 23 October 2024; Issue published 03 January 2025
Abstract
The rapid development of short video platforms poses new challenges for traditional recommendation systems. Recommender systems typically depend on two types of user behavior feedback to construct user interest profiles: explicit feedback (interactive behavior), which significantly influences users’ short-term interests, and implicit feedback (viewing time), which substantially affects their long-term interests. However, the previous model fails to distinguish between these two feedback methods, leading it to predict only the overall preferences of users based on extensive historical behavior sequences. Consequently, it cannot differentiate between users’ long-term and short-term interests, resulting in low accuracy in describing users’ interest states and predicting the evolution of their interests. This paper introduces a video recommendation model called CAT-MF Rec (Cross Attention Transformer-Mixed Feedback Recommendation) designed to differentiate between explicit and implicit user feedback within the DIEN (Deep Interest Evolution Network) framework. This study emphasizes the separate learning of the two types of behavioral feedback, effectively integrating them through the cross-attention mechanism. Additionally, it leverages the long sequence dependence capabilities of Transformer technology to accurately construct user interest profiles and predict the evolution of user interests. Experimental results indicate that CAT-MF Rec significantly outperforms existing recommendation methods across various performance indicators. This advancement offers new theoretical and practical insights for the development of video recommendations, particularly in addressing complex and dynamic user behavior patterns.Keywords
Cite This Article
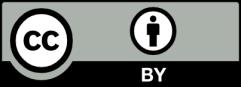
This work is licensed under a Creative Commons Attribution 4.0 International License , which permits unrestricted use, distribution, and reproduction in any medium, provided the original work is properly cited.