Open Access
ARTICLE
DecMamba: Mamba Utilizing Series Decomposition for Multivariate Time Series Forecasting
Communication and Network Key Laboratory, Dalian University, Dalian, 116622, China
* Corresponding Author: Jianxin Feng. Email:
Computers, Materials & Continua 2025, 82(1), 1049-1068. https://doi.org/10.32604/cmc.2024.058374
Received 11 September 2024; Accepted 22 October 2024; Issue published 03 January 2025
Abstract
Multivariate time series forecasting is widely used in traffic planning, weather forecasting, and energy consumption. Series decomposition algorithms can help models better understand the underlying patterns of the original series to improve the forecasting accuracy of multivariate time series. However, the decomposition kernel of previous decomposition-based models is fixed, and these models have not considered the differences in frequency fluctuations between components. These problems make it difficult to analyze the intricate temporal variations of real-world time series. In this paper, we propose a series decomposition-based Mamba model, DecMamba, to obtain the intricate temporal dependencies and the dependencies among different variables of multivariate time series. A variable-level adaptive kernel combination search module is designed to interact with information on different trends and periods between variables. Two backbone structures are proposed to emphasize the differences in frequency fluctuations of seasonal and trend components. Mamba with superior performance is used instead of a Transformer in backbone structures to capture the dependencies among different variables. A new embedding block is designed to capture the temporal features better, especially for the high-frequency seasonal component whose semantic information is difficult to acquire. A gating mechanism is introduced to the decoder in the seasonal backbone to improve the prediction accuracy. A comparison with ten state-of-the-art models on seven real-world datasets demonstrates that DecMamba can better model the temporal dependencies and the dependencies among different variables, guaranteeing better prediction performance for multivariate time series.Keywords
Cite This Article
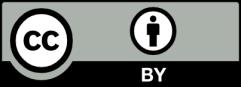
This work is licensed under a Creative Commons Attribution 4.0 International License , which permits unrestricted use, distribution, and reproduction in any medium, provided the original work is properly cited.