Open Access
ARTICLE
Steel Surface Defect Detection Using Learnable Memory Vision Transformer
1 Department of Computer Science and Engineering, BRAC University, Dhaka, 1212, Bangladesh
2 Department of AI and Big Data, Endicott College, Woosong University, Daejeon, 34606, Republic of Korea
* Corresponding Author: Jia Uddin. Email:
# These authors contributed equally to this work
(This article belongs to the Special Issue: Advancements in Machine Fault Diagnosis and Prognosis: Data-Driven Approaches and Autonomous Systems)
Computers, Materials & Continua 2025, 82(1), 499-520. https://doi.org/10.32604/cmc.2025.058361
Received 10 September 2024; Accepted 17 December 2024; Issue published 03 January 2025
Abstract
This study investigates the application of Learnable Memory Vision Transformers (LMViT) for detecting metal surface flaws, comparing their performance with traditional CNNs, specifically ResNet18 and ResNet50, as well as other transformer-based models including Token to Token ViT, ViT without memory, and Parallel ViT. Leveraging a widely-used steel surface defect dataset, the research applies data augmentation and t-distributed stochastic neighbor embedding (t-SNE) to enhance feature extraction and understanding. These techniques mitigated overfitting, stabilized training, and improved generalization capabilities. The LMViT model achieved a test accuracy of 97.22%, significantly outperforming ResNet18 (88.89%) and ResNet50 (88.90%), as well as the Token to Token ViT (88.46%), ViT without memory (87.18), and Parallel ViT (91.03%). Furthermore, LMViT exhibited superior training and validation performance, attaining a validation accuracy of 98.2% compared to 91.0% for ResNet18, 96.0% for ResNet50, and 89.12%, 87.51%, and 91.21% for Token to Token ViT, ViT without memory, and Parallel ViT, respectively. The findings highlight the LMViT’s ability to capture long-range dependencies in images, an area where CNNs struggle due to their reliance on local receptive fields and hierarchical feature extraction. The additional transformer-based models also demonstrate improved performance in capturing complex features over CNNs, with LMViT excelling particularly at detecting subtle and complex defects, which is critical for maintaining product quality and operational efficiency in industrial applications. For instance, the LMViT model successfully identified fine scratches and minor surface irregularities that CNNs often misclassify. This study not only demonstrates LMViT’s potential for real-world defect detection but also underscores the promise of other transformer-based architectures like Token to Token ViT, ViT without memory, and Parallel ViT in industrial scenarios where complex spatial relationships are key. Future research may focus on enhancing LMViT’s computational efficiency for deployment in real-time quality control systems.Keywords
Cite This Article
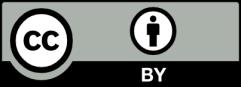
This work is licensed under a Creative Commons Attribution 4.0 International License , which permits unrestricted use, distribution, and reproduction in any medium, provided the original work is properly cited.