Open Access
ARTICLE
DDoS Attack Autonomous Detection Model Based on Multi-Strategy Integrate Zebra Optimization Algorithm
1 School of Information Engineering, Institute of Disaster Prevention, Langfang, 065201, China
2 Langfang Key Laboratory of Network Emergency Protection and Network Security, Langfang, 065201, China
* Corresponding Author: Xiaoying Wang. Email:
(This article belongs to the Special Issue: Security, Privacy, and Robustness for Trustworthy AI Systems)
Computers, Materials & Continua 2025, 82(1), 645-674. https://doi.org/10.32604/cmc.2024.058081
Received 04 September 2024; Accepted 18 October 2024; Issue published 03 January 2025
Abstract
Previous studies have shown that deep learning is very effective in detecting known attacks. However, when facing unknown attacks, models such as Deep Neural Networks (DNN) combined with Long Short-Term Memory (LSTM), Convolutional Neural Networks (CNN) combined with LSTM, and so on are built by simple stacking, which has the problems of feature loss, low efficiency, and low accuracy. Therefore, this paper proposes an autonomous detection model for Distributed Denial of Service attacks, Multi-Scale Convolutional Neural Network-Bidirectional Gated Recurrent Units-Single Headed Attention (MSCNN-BiGRU-SHA), which is based on a Multi-strategy Integrated Zebra Optimization Algorithm (MI-ZOA). The model undergoes training and testing with the CICDDoS2019 dataset, and its performance is evaluated on a new GINKS2023 dataset. The hyperparameters for Conv_filter and GRU_unit are optimized using the Multi-strategy Integrated Zebra Optimization Algorithm (MI-ZOA). The experimental results show that the test accuracy of the MSCNN-BiGRU-SHA model based on the MI-ZOA proposed in this paper is as high as 0.9971 in the CICDDoS2019 dataset. The evaluation accuracy of the new dataset GINKS2023 created in this paper is 0.9386. Compared to the MSCNN-BiGRU-SHA model based on the Zebra Optimization Algorithm (ZOA), the detection accuracy on the GINKS2023 dataset has improved by 5.81%, precision has increased by 1.35%, the recall has improved by 9%, and the F1 score has increased by 5.55%. Compared to the MSCNN-BiGRU-SHA models developed using Grid Search, Random Search, and Bayesian Optimization, the MSCNN-BiGRU-SHA model optimized with the MI-ZOA exhibits better performance in terms of accuracy, precision, recall, and F1 score.Keywords
Cite This Article
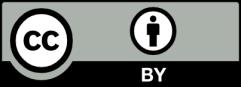
This work is licensed under a Creative Commons Attribution 4.0 International License , which permits unrestricted use, distribution, and reproduction in any medium, provided the original work is properly cited.