Open Access
ARTICLE
A Lightweight Multiscale Feature Fusion Network for Solar Cell Defect Detection
1 School of Mechanical Engineering, Guizhou University, Guiyang, 550025, China
2 School of Computer Science and Technology, Beijing Jiaotong University, Beijing, 100044, China
* Corresponding Author: Linna Zhang. Email:
Computers, Materials & Continua 2025, 82(1), 521-542. https://doi.org/10.32604/cmc.2024.058063
Received 03 September 2024; Accepted 14 October 2024; Issue published 03 January 2025
Abstract
Solar cell defect detection is crucial for quality inspection in photovoltaic power generation modules. In the production process, defect samples occur infrequently and exhibit random shapes and sizes, which makes it challenging to collect defective samples. Additionally, the complex surface background of polysilicon cell wafers complicates the accurate identification and localization of defective regions. This paper proposes a novel Lightweight Multi-scale Feature Fusion network (LMFF) to address these challenges. The network comprises a feature extraction network, a multi-scale feature fusion module (MFF), and a segmentation network. Specifically, a feature extraction network is proposed to obtain multi-scale feature outputs, and a multi-scale feature fusion module (MFF) is used to fuse multi-scale feature information effectively. In order to capture finer-grained multi-scale information from the fusion features, we propose a multi-scale attention module (MSA) in the segmentation network to enhance the network’s ability for small target detection. Moreover, depthwise separable convolutions are introduced to construct depthwise separable residual blocks (DSR) to reduce the model’s parameter number. Finally, to validate the proposed method’s defect segmentation and localization performance, we constructed three solar cell defect detection datasets: SolarCells, SolarCells-S, and PVEL-S. SolarCells and SolarCells-S are monocrystalline silicon datasets, and PVEL-S is a polycrystalline silicon dataset. Experimental results show that the IOU of our method on these three datasets can reach 68.5%, 51.0%, and 92.7%, respectively, and the F1-Score can reach 81.3%, 67.5%, and 96.2%, respectively, which surpasses other commonly used methods and verifies the effectiveness of our LMFF network.Keywords
Cite This Article
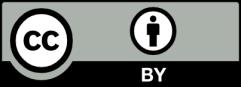
This work is licensed under a Creative Commons Attribution 4.0 International License , which permits unrestricted use, distribution, and reproduction in any medium, provided the original work is properly cited.