Open Access
ARTICLE
GFRF R-CNN: Object Detection Algorithm for Transmission Lines
1 Shanghai Advanced Research Institute, Chinese Academy of Sciences, Shanghai, 201210, China
2 University of Chinese Academy of Sciences, Beijing, 100049, China
3 Jingwei Textile Machinery Co., Ltd., Beijing, 100176, China
* Corresponding Author: Jianfeng Yu. Email:
(This article belongs to the Special Issue: Advances in Object Detection: Methods and Applications)
Computers, Materials & Continua 2025, 82(1), 1439-1458. https://doi.org/10.32604/cmc.2024.057797
Received 27 August 2024; Accepted 30 October 2024; Issue published 03 January 2025
Abstract
To maintain the reliability of power systems, routine inspections using drones equipped with advanced object detection algorithms are essential for preempting power-related issues. The increasing resolution of drone-captured images has posed a challenge for traditional target detection methods, especially in identifying small objects in high-resolution images. This study presents an enhanced object detection algorithm based on the Faster Region-based Convolutional Neural Network (Faster R-CNN) framework, specifically tailored for detecting small-scale electrical components like insulators, shock hammers, and screws in transmission line. The algorithm features an improved backbone network for Faster R-CNN, which significantly boosts the feature extraction network’s ability to detect fine details. The Region Proposal Network is optimized using a method of guided feature refinement (GFR), which achieves a balance between accuracy and speed. The incorporation of Generalized Intersection over Union (GIOU) and Region of Interest (ROI) Align further refines the model’s accuracy. Experimental results demonstrate a notable improvement in mean Average Precision, reaching 89.3%, an 11.1% increase compared to the standard Faster R-CNN. This highlights the effectiveness of the proposed algorithm in identifying electrical components in high-resolution aerial images.Keywords
Cite This Article
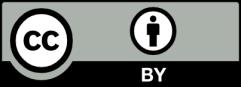
This work is licensed under a Creative Commons Attribution 4.0 International License , which permits unrestricted use, distribution, and reproduction in any medium, provided the original work is properly cited.