Open Access
ARTICLE
Loss Aware Feature Attention Mechanism for Class and Feature Imbalance Issue
1 College of Information and Communication Engineering, Communication University of China, Beijing, 100024, China
2 State Key Laboratory of Media Convergence and Communication, Communication University of China, Beijing, 100024, China
* Corresponding Author: Fulian Yin. Email:
Computers, Materials & Continua 2025, 82(1), 751-775. https://doi.org/10.32604/cmc.2024.057606
Received 22 August 2024; Accepted 22 October 2024; Issue published 03 January 2025
Abstract
In the Internet era, recommendation systems play a crucial role in helping users find relevant information from large datasets. Class imbalance is known to severely affect data quality, and therefore reduce the performance of recommendation systems. Due to the imbalance, machine learning algorithms tend to classify inputs into the positive (majority) class every time to achieve high prediction accuracy. Imbalance can be categorized such as by features and classes, but most studies consider only class imbalance. In this paper, we propose a recommendation system that can integrate multiple networks to adapt to a large number of imbalanced features and can deal with highly skewed and imbalanced datasets through a loss function. We propose a loss aware feature attention mechanism (LAFAM) to solve the issue of feature imbalance. The network incorporates an attention mechanism and uses multiple sub-networks to classify and learn features. For better results, the network can learn the weights of sub-networks and assign higher weights to important features. We propose suppression loss to address class imbalance, which favors negative loss by penalizing positive loss, and pays more attention to sample points near the decision boundary. Experiments on two large-scale datasets verify that the performance of the proposed system is greatly improved compared to baseline methods.Keywords
Cite This Article
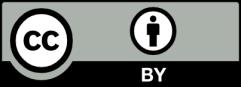
This work is licensed under a Creative Commons Attribution 4.0 International License , which permits unrestricted use, distribution, and reproduction in any medium, provided the original work is properly cited.