Open Access
ARTICLE
Joint Feature Encoding and Task Alignment Mechanism for Emotion-Cause Pair Extraction
College of Computer and Control Engineering, Northeast Forestry University, Harbin, 150040, China
* Corresponding Author: Didi Sun. Email:
(This article belongs to the Special Issue: Next-Generation Techniques and Applications on Opinion Mining and Affective Computing)
Computers, Materials & Continua 2025, 82(1), 1069-1086. https://doi.org/10.32604/cmc.2024.057349
Received 15 August 2024; Accepted 30 October 2024; Issue published 03 January 2025
Abstract
With the rapid expansion of social media, analyzing emotions and their causes in texts has gained significant importance. Emotion-cause pair extraction enables the identification of causal relationships between emotions and their triggers within a text, facilitating a deeper understanding of expressed sentiments and their underlying reasons. This comprehension is crucial for making informed strategic decisions in various business and societal contexts. However, recent research approaches employing multi-task learning frameworks for modeling often face challenges such as the inability to simultaneously model extracted features and their interactions, or inconsistencies in label prediction between emotion-cause pair extraction and independent assistant tasks like emotion and cause extraction. To address these issues, this study proposes an emotion-cause pair extraction methodology that incorporates joint feature encoding and task alignment mechanisms. The model consists of two primary components: First, joint feature encoding simultaneously generates features for emotion-cause pairs and clauses, enhancing feature interactions between emotion clauses, cause clauses, and emotion-cause pairs. Second, the task alignment technique is applied to reduce the labeling distance between emotion-cause pair extraction and the two assistant tasks, capturing deep semantic information interactions among tasks. The proposed method is evaluated on a Chinese benchmark corpus using 10-fold cross-validation, assessing key performance metrics such as precision, recall, and F1 score. Experimental results demonstrate that the model achieves an F1 score of 76.05%, surpassing the state-of-the-art by 1.03%. The proposed model exhibits significant improvements in emotion-cause pair extraction (ECPE) and cause extraction (CE) compared to existing methods, validating its effectiveness. This research introduces a novel approach based on joint feature encoding and task alignment mechanisms, contributing to advancements in emotion-cause pair extraction. However, the study’s limitation lies in the data sources, potentially restricting the generalizability of the findings.Keywords
Cite This Article
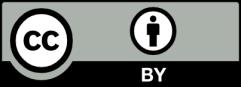
This work is licensed under a Creative Commons Attribution 4.0 International License , which permits unrestricted use, distribution, and reproduction in any medium, provided the original work is properly cited.