Open Access
ARTICLE
A Generative Model-Based Network Framework for Ecological Data Reconstruction
1 School of Electronic and Information Engineering, University of Science and Technology Liaoning, Anshan, 114051, China
2 School of Computer Science and Software Engineering, University of Science and Technology Liaoning, Anshan, 114051, China
* Corresponding Author: Zhao Zhang. Email:
Computers, Materials & Continua 2025, 82(1), 929-948. https://doi.org/10.32604/cmc.2024.057319
Received 14 August 2024; Accepted 24 October 2024; Issue published 03 January 2025
Abstract
This study examines the effectiveness of artificial intelligence techniques in generating high-quality environmental data for species introductory site selection systems. Combining Strengths, Weaknesses, Opportunities, Threats (SWOT) analysis data with Variation Autoencoder (VAE) and Generative Adversarial Network (GAN) the network framework model (SAE-GAN), is proposed for environmental data reconstruction. The model combines two popular generative models, GAN and VAE, to generate features conditional on categorical data embedding after SWOT Analysis. The model is capable of generating features that resemble real feature distributions and adding sample factors to more accurately track individual sample data. Reconstructed data is used to retain more semantic information to generate features. The model was applied to species in Southern California, USA, citing SWOT analysis data to train the model. Experiments show that the model is capable of integrating data from more comprehensive analyses than traditional methods and generating high-quality reconstructed data from them, effectively solving the problem of insufficient data collection in development environments. The model is further validated by the Technique for Order Preference by Similarity to an Ideal Solution (TOPSIS) classification assessment commonly used in the environmental data domain. This study provides a reliable and rich source of training data for species introduction site selection systems and makes a significant contribution to ecological and sustainable development.Keywords
Cite This Article
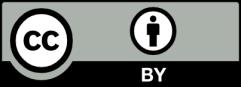
This work is licensed under a Creative Commons Attribution 4.0 International License , which permits unrestricted use, distribution, and reproduction in any medium, provided the original work is properly cited.