Open Access
ARTICLE
A Hybrid Approach for Pavement Crack Detection Using Mask R-CNN and Vision Transformer Model
Faculty of Engineering, China University of Geosciences, Wuhan, 430074, China
* Corresponding Author: Li Wu. Email:
(This article belongs to the Special Issue: Industrial Big Data and Artificial Intelligence-Driven Intelligent Perception, Maintenance, and Decision Optimization in Industrial Systems)
Computers, Materials & Continua 2025, 82(1), 561-577. https://doi.org/10.32604/cmc.2024.057213
Received 11 August 2024; Accepted 14 October 2024; Issue published 03 January 2025
Abstract
Detecting pavement cracks is critical for road safety and infrastructure management. Traditional methods, relying on manual inspection and basic image processing, are time-consuming and prone to errors. Recent deep-learning (DL) methods automate crack detection, but many still struggle with variable crack patterns and environmental conditions. This study aims to address these limitations by introducing the MaskerTransformer, a novel hybrid deep learning model that integrates the precise localization capabilities of Mask Region-based Convolutional Neural Network (Mask R-CNN) with the global contextual awareness of Vision Transformer (ViT). The research focuses on leveraging the strengths of both architectures to enhance segmentation accuracy and adaptability across different pavement conditions. We evaluated the performance of the MaskerTransformer against other state-of-the-art models such as U-Net, Transformer U-Net (TransUNet), U-Net Transformer (UNETr), Swin U-Net Transformer (Swin-UNETr), You Only Look Once version 8 (YoloV8), and Mask R-CNN using two benchmark datasets: Crack500 and DeepCrack. The findings reveal that the MaskerTransformer significantly outperforms the existing models, achieving the highest Dice Similarity Coefficient (DSC), precision, recall, and F1-Score across both datasets. Specifically, the model attained a DSC of 80.04% on Crack500 and 91.37% on DeepCrack, demonstrating superior segmentation accuracy and reliability. The high precision and recall rates further substantiate its effectiveness in real-world applications, suggesting that the MaskerTransformer can serve as a robust tool for automated pavement crack detection, potentially replacing more traditional methods.Keywords
Cite This Article
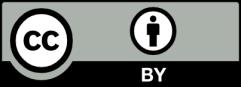
This work is licensed under a Creative Commons Attribution 4.0 International License , which permits unrestricted use, distribution, and reproduction in any medium, provided the original work is properly cited.