Open Access
ARTICLE
A Multi-Objective Particle Swarm Optimization Algorithm Based on Decomposition and Multi-Selection Strategy
1 School of Computer Science, Shaanxi Normal University, Xi’an, 710119, China
2 Fujian Provincial Key Laboratory of Big Data Mining and Applications, Fujian University of Technology, Fuzhou, 350118, China
3 Information Construction and Management Center and Institute of Artificial Intelligence and Educational New Productivity, Ningxia Normal University, Guyuan, 756099, China
* Corresponding Author: Cai Dai. Email:
Computers, Materials & Continua 2025, 82(1), 997-1026. https://doi.org/10.32604/cmc.2024.057168
Received 09 August 2024; Accepted 25 October 2024; Issue published 03 January 2025
Abstract
The multi-objective particle swarm optimization algorithm (MOPSO) is widely used to solve multi-objective optimization problems. In the article, a multi-objective particle swarm optimization algorithm based on decomposition and multi-selection strategy is proposed to improve the search efficiency. First, two update strategies based on decomposition are used to update the evolving population and external archive, respectively. Second, a multi-selection strategy is designed. The first strategy is for the subspace without a non-dominated solution. Among the neighbor particles, the particle with the smallest penalty-based boundary intersection value is selected as the global optimal solution and the particle far away from the search particle and the global optimal solution is selected as the personal optimal solution to enhance global search. The second strategy is for the subspace with a non-dominated solution. In the neighbor particles, two particles are randomly selected, one as the global optimal solution and the other as the personal optimal solution, to enhance local search. The third strategy is for Pareto optimal front (PF) discontinuity, which is identified by the cumulative number of iterations of the subspace without non-dominated solutions. In the subsequent iteration, a new probability distribution is used to select from the remaining subspaces to search. Third, an adaptive inertia weight update strategy based on the dominated degree is designed to further improve the search efficiency. Finally, the proposed algorithm is compared with five multi-objective particle swarm optimization algorithms and five multi-objective evolutionary algorithms on 22 test problems. The results show that the proposed algorithm has better performance.Keywords
Cite This Article
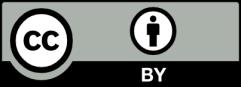
This work is licensed under a Creative Commons Attribution 4.0 International License , which permits unrestricted use, distribution, and reproduction in any medium, provided the original work is properly cited.