Open Access
ARTICLE
Unmasking Social Robots’ Camouflage: A GNN-Random Forest Framework for Enhanced Detection
1 School of Data Science and Intelligent Media, Communication University of China, Beijing, 100024, China
2 School of Computer and Cyber Sciences, Communication University of China, Beijing, 100024, China
3 Institute of Communication Studies, Communication University of China, Beijing, 100024, China
4 State Key Laboratory of Media Convergence and Communication, Communication University of China, Beijing, 100024, China
* Corresponding Author: Weijian Fan. Email:
Computers, Materials & Continua 2025, 82(1), 467-483. https://doi.org/10.32604/cmc.2024.056930
Received 02 August 2024; Accepted 12 October 2024; Issue published 03 January 2025
Abstract
The proliferation of robot accounts on social media platforms has posed a significant negative impact, necessitating robust measures to counter network anomalies and safeguard content integrity. Social robot detection has emerged as a pivotal yet intricate task, aimed at mitigating the dissemination of misleading information. While graph-based approaches have attained remarkable performance in this realm, they grapple with a fundamental limitation: the homogeneity assumption in graph convolution allows social robots to stealthily evade detection by mingling with genuine human profiles. To unravel this challenge and thwart the camouflage tactics, this work proposed an innovative social robot detection framework based on enhanced HOmogeneity and Random Forest (HORFBot). At the core of HORFBot lies a homogeneous graph enhancement strategy, intricately woven with edge-removal techniques, to meticulously dissect the graph into multiple revealing subgraphs. Subsequently, leveraging the power of contrastive learning, the proposed methodology meticulously trains multiple graph convolutional networks, each honed to discern nuances within these tailored subgraphs. The culminating stage involves the fusion of these feature-rich base classifiers, harmoniously aggregating their insights to produce a comprehensive detection outcome. Extensive experiments on three social robot detection datasets have shown that this method effectively improves the accuracy of social robot detection and outperforms comparative methods.Keywords
Cite This Article
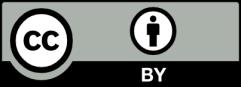
This work is licensed under a Creative Commons Attribution 4.0 International License , which permits unrestricted use, distribution, and reproduction in any medium, provided the original work is properly cited.