Open Access
ARTICLE
Text-Image Feature Fine-Grained Learning for Joint Multimodal Aspect-Based Sentiment Analysis
1 School of Data and Target Engineering, Information Engineering University, Zhengzhou, 450001, China
2 Information Engineering Department, Liaoning Provincial College of Communications, Shenyang, 110122, China
3 School of Computer and Artificial Intelligence, Zhengzhou University, Zhengzhou, 450000, China
* Corresponding Author: Gang Zhou. Email:
Computers, Materials & Continua 2025, 82(1), 279-305. https://doi.org/10.32604/cmc.2024.055943
Received 10 July 2024; Accepted 05 October 2024; Issue published 03 January 2025
Abstract
Joint Multimodal Aspect-based Sentiment Analysis (JMASA) is a significant task in the research of multimodal fine-grained sentiment analysis, which combines two subtasks: Multimodal Aspect Term Extraction (MATE) and Multimodal Aspect-oriented Sentiment Classification (MASC). Currently, most existing models for JMASA only perform text and image feature encoding from a basic level, but often neglect the in-depth analysis of unimodal intrinsic features, which may lead to the low accuracy of aspect term extraction and the poor ability of sentiment prediction due to the insufficient learning of intra-modal features. Given this problem, we propose a Text-Image Feature Fine-grained Learning (TIFFL) model for JMASA. First, we construct an enhanced adjacency matrix of word dependencies and adopt graph convolutional network to learn the syntactic structure features for text, which addresses the context interference problem of identifying different aspect terms. Then, the adjective-noun pairs extracted from image are introduced to enable the semantic representation of visual features more intuitive, which addresses the ambiguous semantic extraction problem during image feature learning. Thereby, the model performance of aspect term extraction and sentiment polarity prediction can be further optimized and enhanced. Experiments on two Twitter benchmark datasets demonstrate that TIFFL achieves competitive results for JMASA, MATE and MASC, thus validating the effectiveness of our proposed methods.Keywords
Cite This Article
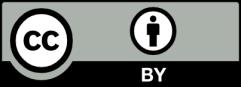
This work is licensed under a Creative Commons Attribution 4.0 International License , which permits unrestricted use, distribution, and reproduction in any medium, provided the original work is properly cited.