Open Access
ARTICLE
Occluded Gait Emotion Recognition Based on Multi-Scale Suppression Graph Convolutional Network
1 Beijing Key Laboratory of Information Service Engineering, Beijing Union University, Beijing, 100101, China
2 College of Smart City, Beijing Union University, Beijing, 100101, China
* Corresponding Author: Ning He. Email:
Computers, Materials & Continua 2025, 82(1), 1255-1276. https://doi.org/10.32604/cmc.2024.055732
Received 05 July 2024; Accepted 07 October 2024; Issue published 03 January 2025
Abstract
In recent years, gait-based emotion recognition has been widely applied in the field of computer vision. However, existing gait emotion recognition methods typically rely on complete human skeleton data, and their accuracy significantly declines when the data is occluded. To enhance the accuracy of gait emotion recognition under occlusion, this paper proposes a Multi-scale Suppression Graph Convolutional Network (MS-GCN). The MS-GCN consists of three main components: Joint Interpolation Module (JI Moudle), Multi-scale Temporal Convolution Network (MS-TCN), and Suppression Graph Convolutional Network (SGCN). The JI Module completes the spatially occluded skeletal joints using the (K-Nearest Neighbors) KNN interpolation method. The MS-TCN employs convolutional kernels of various sizes to comprehensively capture the emotional information embedded in the gait, compensating for the temporal occlusion of gait information. The SGCN extracts more non-prominent human gait features by suppressing the extraction of key body part features, thereby reducing the negative impact of occlusion on emotion recognition results. The proposed method is evaluated on two comprehensive datasets: Emotion-Gait, containing 4227 real gaits from sources like BML, ICT-Pollick, and ELMD, and 1000 synthetic gaits generated using STEP-Gen technology, and ELMB, consisting of 3924 gaits, with 1835 labeled with emotions such as “Happy,” “Sad,” “Angry,” and “Neutral.” On the standard datasets Emotion-Gait and ELMB, the proposed method achieved accuracies of 0.900 and 0.896, respectively, attaining performance comparable to other state-of-the-art methods. Furthermore, on occlusion datasets, the proposed method significantly mitigates the performance degradation caused by occlusion compared to other methods, the accuracy is significantly higher than that of other methods.Keywords
Cite This Article
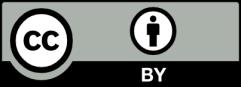
This work is licensed under a Creative Commons Attribution 4.0 International License , which permits unrestricted use, distribution, and reproduction in any medium, provided the original work is properly cited.