Open Access
ARTICLE
Robust Network Security: A Deep Learning Approach to Intrusion Detection in IoT
Department of Computer Science, Princess Sumaya University of Technology, Amman, 1196, Jordan
* Corresponding Author: Ammar Odeh. Email:
(This article belongs to the Special Issue: Fortifying the Foundations: IoT Intrusion Detection Systems in Cloud-Edge-End Architecture)
Computers, Materials & Continua 2024, 81(3), 4149-4169. https://doi.org/10.32604/cmc.2024.058052
Received 03 September 2024; Accepted 05 November 2024; Issue published 19 December 2024
Abstract
The proliferation of Internet of Things (IoT) technology has exponentially increased the number of devices interconnected over networks, thereby escalating the potential vectors for cybersecurity threats. In response, this study rigorously applies and evaluates deep learning models—namely Convolutional Neural Networks (CNN), Autoencoders, and Long Short-Term Memory (LSTM) networks—to engineer an advanced Intrusion Detection System (IDS) specifically designed for IoT environments. Utilizing the comprehensive UNSW-NB15 dataset, which encompasses 49 distinct features representing varied network traffic characteristics, our methodology focused on meticulous data preprocessing including cleaning, normalization, and strategic feature selection to enhance model performance. A robust comparative analysis highlights the CNN model’s outstanding performance, achieving an accuracy of 99.89%, precision of 99.90%, recall of 99.88%, and an F1 score of 99.89% in binary classification tasks, outperforming other evaluated models significantly. These results not only confirm the superior detection capabilities of CNNs in distinguishing between benign and malicious network activities but also illustrate the model’s effectiveness in multiclass classification tasks, addressing various attack vectors prevalent in IoT setups. The empirical findings from this research demonstrate deep learning’s transformative potential in fortifying network security infrastructures against sophisticated cyber threats, providing a scalable, high-performance solution that enhances security measures across increasingly complex IoT ecosystems. This study’s outcomes are critical for security practitioners and researchers focusing on the next generation of cyber defense mechanisms, offering a data-driven foundation for future advancements in IoT security strategies.Keywords
Cite This Article
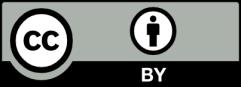
This work is licensed under a Creative Commons Attribution 4.0 International License , which permits unrestricted use, distribution, and reproduction in any medium, provided the original work is properly cited.