Open Access
ARTICLE
Evaluation of Modern Generative Networks for EchoCG Image Generation
1 Department of Cybersecurity, Information Processing and Storage, Satbayev University, Almaty, 050000, Kazakhstan
2 Department of Mathematical Computer Modeling, International IT University, Almaty, 050000, Kazakhstan
3 Department of Information Systems, International IT University, Almaty, 050000, Kazakhstan
* Corresponding Author: Sabina Rakhmetulayeva. Email:
Computers, Materials & Continua 2024, 81(3), 4503-4523. https://doi.org/10.32604/cmc.2024.057974
Received 01 September 2024; Accepted 11 November 2024; Issue published 19 December 2024
Abstract
The applications of machine learning (ML) in the medical domain are often hindered by the limited availability of high-quality data. To address this challenge, we explore the synthetic generation of echocardiography images (echoCG) using state-of-the-art generative models. We conduct a comprehensive evaluation of three prominent methods: Cycle-consistent generative adversarial network (CycleGAN), Contrastive Unpaired Translation (CUT), and Stable Diffusion 1.5 with Low-Rank Adaptation (LoRA). Our research presents the data generation methodology, image samples, and evaluation strategy, followed by an extensive user study involving licensed cardiologists and surgeons who assess the perceived quality and medical soundness of the generated images. Our findings indicate that Stable Diffusion outperforms both CycleGAN and CUT in generating images that are nearly indistinguishable from real echoCG images, making it a promising tool for augmenting medical datasets. However, we also identify limitations in the synthetic images generated by CycleGAN and CUT, which are easily distinguishable as non-realistic by medical professionals. This study highlights the potential of diffusion models in medical imaging and their applicability in addressing data scarcity, while also outlining the areas for future improvement.Graphic Abstract
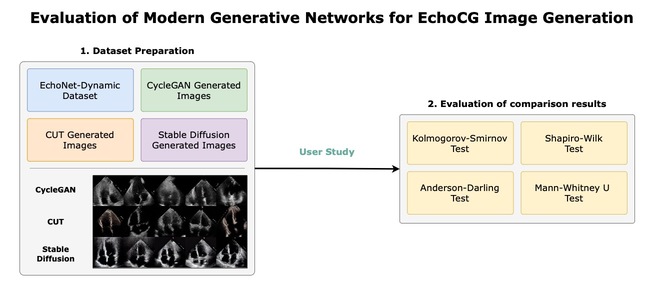
Keywords
Cite This Article
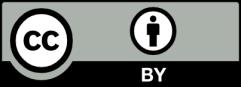
This work is licensed under a Creative Commons Attribution 4.0 International License , which permits unrestricted use, distribution, and reproduction in any medium, provided the original work is properly cited.