Open Access
ARTICLE
Uncovering Causal Relationships for Debiased Repost Prediction Using Deep Generative Models
1 School of Computer Science and Engineering, Southeast University, Nanjing, 211189, China
2 Department of Media and Communication, City University of Hong Kong, Hong Kong SAR, 999077, China
* Corresponding Author: Xiao Fan Liu. Email:
Computers, Materials & Continua 2024, 81(3), 4551-4573. https://doi.org/10.32604/cmc.2024.057714
Received 26 August 2024; Accepted 14 November 2024; Issue published 19 December 2024
Abstract
Microblogging platforms like X (formerly Twitter) and Sina Weibo have become key channels for spreading information online. Accurately predicting information spread, such as users’ reposting activities, is essential for applications including content recommendation and analyzing public sentiment. Current advanced models rely on deep representation learning to extract features from various inputs, such as users’ social connections and repost history, to forecast reposting behavior. Nonetheless, these models frequently ignore intrinsic confounding factors, which may cause the models to capture spurious relationships, ultimately impacting prediction performance. To address this limitation, we propose a novel Debiased Reposting Prediction model (DRP). Our model mitigates the influence of confounding variables by incorporating intervention operations from causal inference, enabling it to learn the causal associations between features and user reposting behavior. Specifically, we introduce a memory network within DRP to enhance the model’s perception of confounder distributions. This network aggregates and learns confounding information dispersed across different training data batches by optimizing the reconstruction loss. Furthermore, recognizing the challenge of acquiring prior knowledge of causal graphs, which is crucial for causal inference, we develop a causal discovery module within DRP (CD-DRP). This module allows the model to autonomously uncover the causal graph of feature variables by analyzing microblogging data. Experimental results on multiple real-world datasets demonstrate that our proposed method effectively uncovers causal relationships between variables, exhibits strong time efficiency, and outperforms state-of-the-art models in prediction performance (improved by 2.54%) and overfitting reduction (by 7.44%).Keywords
Cite This Article
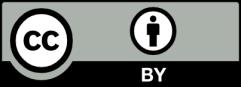
This work is licensed under a Creative Commons Attribution 4.0 International License , which permits unrestricted use, distribution, and reproduction in any medium, provided the original work is properly cited.