Open Access
ARTICLE
A Hybrid WSVM-Levy Approach for Energy-Efficient Manufacturing Using Big Data and IoT
1 School of Science, Engineering and Environment, University of Salford, Manchester, M6 8HD, UK
2 University Centre for Research and Development, Chandigarh University, Mohali, 140307, India
3 Management Information System Department, College of Business Administration, King Saud University, Riyadh, 11495, Saudi Arabia
4 Department of Computer Science and Engineering, JAIN (Deemed-to-be University), Bangalore, 560030, India
5 Department of Computer Science and Engineering, IES College of Technology, Bhopal, 462044, India
* Corresponding Author: Surbhi Bhatia Khan. Email:
Computers, Materials & Continua 2024, 81(3), 4895-4914. https://doi.org/10.32604/cmc.2024.057585
Received 21 August 2024; Accepted 11 November 2024; Issue published 19 December 2024
Abstract
In Intelligent Manufacturing, Big Data and industrial information enable enterprises to closely monitor and respond to precise changes in both internal processes and external environmental factors, ensuring more informed decision-making and adaptive system management. It also promotes decision making and provides scientific analysis to enhance the efficiency of the operation, cost reduction, maximizing the process of production and so on. Various methods are employed to enhance productivity, yet achieving sustainable manufacturing remains a complex challenge that requires careful consideration. This study aims to develop a methodology for effective manufacturing sustainability by proposing a novel Hybrid Weighted Support Vector-based Lévy flight (HWS-LF) algorithm. The objective of the HWS-LF method is to improve the environmental, economic, and social aspects of manufacturing processes. In this approach, Support Vector Machines (SVM) are used to classify data points by identifying the optimal hyperplane to separate different classes, thereby supporting predictive maintenance and quality control in manufacturing. Random Forest is applied to boost efficiency, resource allocation, and production optimization. A Weighted Average Ensemble technique is employed to combine predictions from multiple models, assigning different weights to ensure an accurate system for evaluating manufacturing performance. Additionally, Lévy flight Optimization is incorporated to enhance the performance of the HWS-LF method further. The method’s effectiveness is assessed using various evaluation metrics, including accuracy, precision, recall, F1-score, and specificity. Results show that the proposed HWS-LF method outperforms other state-of-the-art techniques, demonstrating superior productivity and system performance.Keywords
Cite This Article
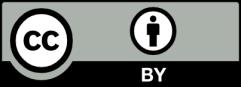
This work is licensed under a Creative Commons Attribution 4.0 International License , which permits unrestricted use, distribution, and reproduction in any medium, provided the original work is properly cited.