Open Access
ARTICLE
An Improved YOLO Detection Approach for Pinpointing Cucumber Diseases and Pests
1 Department of IT Convergence Engineering, University of Kyungnam, Changwon, 51767, Republic of Korea
2 Department of Computer Engineering, University of Kyungnam, Changwon, 51767, Republic of Korea
3 College of Computer Science and Technology, Beihua University, Jilin, 132013, China
* Corresponding Author: Sang-Yong Rhee. Email:
Computers, Materials & Continua 2024, 81(3), 3989-4014. https://doi.org/10.32604/cmc.2024.057473
Received 19 August 2024; Accepted 26 October 2024; Issue published 19 December 2024
Abstract
In complex agricultural environments, cucumber disease identification is confronted with challenges like symptom diversity, environmental interference, and poor detection accuracy. This paper presents the DM-YOLO model, which is an enhanced version of the YOLOv8 framework designed to enhance detection accuracy for cucumber diseases. Traditional detection models have a tough time identifying small-scale and overlapping symptoms, especially when critical features are obscured by lighting variations, occlusion, and background noise. The proposed DM-YOLO model combines three innovative modules to enhance detection performance in a collective way. First, the MultiCat module employs a multi-scale feature processing strategy with adaptive pooling, which decomposes input features into large, medium, and small scales. This approach ensures that high-level features are extracted and fused effectively, effectively improving the detection of smaller and complex patterns that are often missed by traditional methods. Second, the ADC2f module incorporates an attention mechanism and deep separable convolution, which allows the model to focus on the most relevant regions of the input features while reducing computational load. The identification and localization of diseases like downy mildew and powdery mildew can be enhanced by this combination in conditions of lighting changes and occlusion. Finally, the C2fe module introduces a Global Context Block that uses attention mechanisms to emphasize essential regions while suppressing those that are not relevant. This design enables the model to capture more contextual information, which improves detection performance in complex backgrounds and small-object scenarios. A custom cucumber disease dataset and the PlantDoc dataset were used for thorough evaluations. Experimental results showed that DM-YOLO achieved a mean Average Precision (mAP50) improvement of 1.2%p on the custom dataset and 3.2%p on the PlantDoc dataset over the baseline YOLOv8. These results highlight the model’s enhanced ability to detect small-scale and overlapping disease symptoms, demonstrating its effectiveness and robustness in diverse agricultural monitoring environments. Compared to the original algorithm, the improved model shows significant progress and demonstrates strong competitiveness when compared to other advanced object detection models.Keywords
Cite This Article
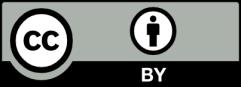
This work is licensed under a Creative Commons Attribution 4.0 International License , which permits unrestricted use, distribution, and reproduction in any medium, provided the original work is properly cited.