Open Access
ARTICLE
Adjusted Reasoning Module for Deep Visual Question Answering Using Vision Transformer
1 Department of Information Technology, Satya Wacana Christian University, Salatiga, 50711, Indonesia
2 Department of Information Systems, Satya Wacana Christian University, Salatiga, 50711, Indonesia
3 School of Information Technology, Deakin University, Burwood, VIC 3125, Australia
4 Department of Marketing and Logistics Management, Chaoyang University of Technology, Taichung City, 413310, Taiwan
* Corresponding Author: Abbott Po Shun Chen. Email:
Computers, Materials & Continua 2024, 81(3), 4195-4216. https://doi.org/10.32604/cmc.2024.057453
Received 18 August 2024; Accepted 01 November 2024; Issue published 19 December 2024
Abstract
Visual Question Answering (VQA) is an interdisciplinary artificial intelligence (AI) activity that integrates computer vision and natural language processing. Its purpose is to empower machines to respond to questions by utilizing visual information. A VQA system typically takes an image and a natural language query as input and produces a textual answer as output. One major obstacle in VQA is identifying a successful method to extract and merge textual and visual data. We examine “Fusion” Models that use information from both the text encoder and picture encoder to efficiently perform the visual question-answering challenge. For the transformer model, we utilize BERT and RoBERTa, which analyze textual data. The image encoder designed for processing image data utilizes ViT (Vision Transformer), Deit (Data-efficient Image Transformer), and BeIT (Image Transformers). The reasoning module of VQA was updated and layer normalization was incorporated to enhance the performance outcome of our effort. In comparison to the results of previous research, our proposed method suggests a substantial enhancement in efficacy. Our experiment obtained a 60.4% accuracy with the PathVQA dataset and a 69.2% accuracy with the VizWiz dataset.Keywords
Cite This Article
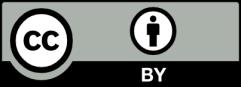
This work is licensed under a Creative Commons Attribution 4.0 International License , which permits unrestricted use, distribution, and reproduction in any medium, provided the original work is properly cited.