Open Access
ARTICLE
A Location Trajectory Privacy Protection Method Based on Generative Adversarial Network and Attention Mechanism
School of Cyber Security, Gansu University of Political Science and Law, Lanzhou, 730070, China
* Corresponding Author: Chen Zhang. Email:
(This article belongs to the Special Issue: Privacy-Preserving Deep Learning and its Advanced Applications)
Computers, Materials & Continua 2024, 81(3), 3781-3804. https://doi.org/10.32604/cmc.2024.057131
Received 08 August 2024; Accepted 30 September 2024; Issue published 19 December 2024
Abstract
User location trajectory refers to the sequence of geographic location information that records the user’s movement or stay within a period of time and is usually used in mobile crowd sensing networks, in which the user participates in the sensing task, the process of sensing data collection faces the problem of privacy leakage. To address the privacy leakage issue of trajectory data during uploading, publishing, and sharing when users use location services on mobile smart group sensing terminal devices, this paper proposes a privacy protection method based on generative adversarial networks and attention mechanisms (BiLS-A-GAN). The method designs a generator attention model, GAttention, and a discriminator attention model, DAttention. In the generator, GAttention, combined with a bidirectional long short-term memory network, more effectively senses contextual information and captures dependencies within sequences. The discriminator uses DAttention and the long short-term memory network to distinguish the authenticity of data. Through continuous interaction between these two models, trajectory data with the statistical characteristics of the original data is generated. This non-original trajectory data can effectively reduce the probability of an attacker’s identification, thereby enhancing the privacy protection of user information. Reliability assessment of the Trajectory-User Linking (TUL) task performed on the real-world semantic trajectory dataset Foursquare NYC, compared with traditional privacy-preserving algorithms that focus only on the privacy enhancement of the data, this approach, while achieving a high level of privacy protection, retains more temporal, spatial, and thematic features from the original trajectory data, to not only guarantee the user’s personal privacy, but also retain the reliability of the information itself in the direction of geographic analysis and other directions, and to achieve the win-win purpose of both data utilization and privacy preservation.Keywords
Cite This Article
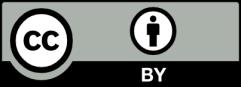
This work is licensed under a Creative Commons Attribution 4.0 International License , which permits unrestricted use, distribution, and reproduction in any medium, provided the original work is properly cited.