Open Access
ARTICLE
Cuckoo Search-Optimized Deep CNN for Enhanced Cyber Security in IoT Networks
1 Department of Computer Science and Information Engineering, Asia University, Taichung, 413, Taiwan
2 Symbiosis Centre for Information Technology (SCIT), Symbiosis International University, Pune, 411057, Maharashtra, India
3 Center for Interdisciplinary Research, University of Petroleum and Energy Studies (UPES), Dehradun, 248007, India
4 University Centre for Research and Development (UCRD), Chandigarh University, Chandigarh, 140413, India
5 Computer Engineering, Ronin Institute, Montclair, NJ 07043, USA
6 Department of Business Administration, Asia University, Taichung, 413, Taiwan
7 Department of Electrical and Computer Engineering, Lebanese American University, Beirut, 1102, Lebanon
8 Management Department, College of Business Administration, Princess Nourah bint Abdulrahman University, Riyadh, 11671, Saudi Arabia
9 Department of Research and Innovation, Insights2Techinfo, Jaipur, 302001, India
10 Department of Computer Science, Faculty of Science, Northern Border University, Arar, 91431, Saudi Arabia
11 Department of Electronic Engineering and Computer Science, Hong Kong Metropolitan University (HKMU), Hong Kong, 518031, China
* Corresponding Author: Brij B. Gupta. Email:
Computers, Materials & Continua 2024, 81(3), 4109-4124. https://doi.org/10.32604/cmc.2024.056476
Received 23 July 2024; Accepted 23 October 2024; Issue published 19 December 2024
Abstract
Phishing attacks seriously threaten information privacy and security within the Internet of Things (IoT) ecosystem. Numerous phishing attack detection solutions have been developed for IoT; however, many of these are either not optimally efficient or lack the lightweight characteristics needed for practical application. This paper proposes and optimizes a lightweight deep-learning model for phishing attack detection. Our model employs a two-fold optimization approach: first, it utilizes the analysis of the variance (ANOVA) F-test to select the optimal features for phishing detection, and second, it applies the Cuckoo Search algorithm to tune the hyperparameters (learning rate and dropout rate) of the deep learning model. Additionally, our model is trained in only five epochs, making it more lightweight than other deep learning (DL) and machine learning (ML) models. The proposed model achieved a phishing detection accuracy of 91%, with a precision of 92% for the ’normal’ class and 91% for the ‘attack’ class. Moreover, the model’s recall and F1-score are 91% for both classes. We also compared our approach with traditional DL/ML models and past literature, demonstrating that our model is more accurate. This study enhances the security of sensitive information and IoT devices by offering a novel and effective approach to phishing detection.Keywords
Cite This Article
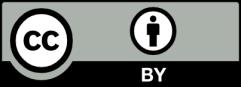
This work is licensed under a Creative Commons Attribution 4.0 International License , which permits unrestricted use, distribution, and reproduction in any medium, provided the original work is properly cited.