Open Access
ARTICLE
A Novel Optimized Deep Convolutional Neural Network for Efficient Seizure Stage Classification
1 Department of Biomedical Engineering, KIT-Kalaignarkarunanidhi Institute of Technology, Coimbatore, 641402, India
2 Department of Computer Sciences and Engineering, Vel Tech Rangarajan Dr. Sagunthala R&D Institute of Science and Technology, Chennai, 600062, India
3 Department of Computer Sciences and Engineering, College of Applied Science, King Saud University, Riyadh, 11543, Saudi Arabia
4 Department of Computer Engineering, College of Computer and Information Sciences, King Saud University, Riyadh, 11543, Saudi Arabia
5 Department of Computer Science and Engineering, Mount Zion College of Engineering and Technology, Pudukkottai, 622507, India
* Corresponding Authors: Umapathi Krishnamoorthy. Email: ; Abdulaziz S. Almazyad. Email:
Computers, Materials & Continua 2024, 81(3), 3903-3926. https://doi.org/10.32604/cmc.2024.055910
Received 10 July 2024; Accepted 11 October 2024; Issue published 19 December 2024
Abstract
Brain signal analysis from electroencephalogram (EEG) recordings is the gold standard for diagnosing various neural disorders especially epileptic seizure. Seizure signals are highly chaotic compared to normal brain signals and thus can be identified from EEG recordings. In the current seizure detection and classification landscape, most models primarily focus on binary classification—distinguishing between seizure and non-seizure states. While effective for basic detection, these models fail to address the nuanced stages of seizures and the intervals between them. Accurate identification of per-seizure or interictal stages and the timing between seizures is crucial for an effective seizure alert system. This granularity is essential for improving patient-specific interventions and developing proactive seizure management strategies. This study addresses this gap by proposing a novel AI-based approach for seizure stage classification using a Deep Convolutional Neural Network (DCNN). The developed model goes beyond traditional binary classification by categorizing EEG recordings into three distinct classes, thus providing a more detailed analysis of seizure stages. To enhance the model’s performance, we have optimized the DCNN using two advanced techniques: the Stochastic Gradient Algorithm (SGA) and the evolutionary Genetic Algorithm (GA). These optimization strategies are designed to fine-tune the model’s accuracy and robustness. Moreover, k-fold cross-validation ensures the model’s reliability and generalizability across different data sets. Trained and validated on the Bonn EEG data sets, the proposed optimized DCNN model achieved a test accuracy of 93.2%, demonstrating its ability to accurately classify EEG signals. In summary, the key advancement of the present research lies in addressing the limitations of existing models by providing a more detailed seizure classification system, thus potentially enhancing the effectiveness of real-time seizure prediction and management systems in clinical settings. With its inherent classification performance, the proposed approach represents a significant step forward in improving patient outcomes through advanced AI techniques.Keywords
Cite This Article
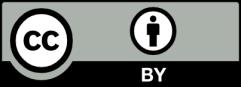
This work is licensed under a Creative Commons Attribution 4.0 International License , which permits unrestricted use, distribution, and reproduction in any medium, provided the original work is properly cited.