Open Access
REVIEW
A Review of Knowledge Graph in Traditional Chinese Medicine: Analysis, Construction, Application and Prospects
1 School of Information Science and Technology, Beijing Forestry University, Beijing, 100083, China
2 National Data Center of Traditional Chinese Medicine, China Academy of Chinese Medical Sciences, Beijing, 100700, China
3 Engineering Research Center for Forestry-oriented Intelligent Information Processing of National Forestry and Grassland Administration, Beijing Forestry University, Beijing, 100083, China
* Corresponding Authors: Dongmei Li. Email: ; Xiaoping Zhang. Email:
Computers, Materials & Continua 2024, 81(3), 3583-3616. https://doi.org/10.32604/cmc.2024.055671
Received 03 July 2024; Accepted 29 September 2024; Issue published 19 December 2024
Abstract
As an advanced data science technology, the knowledge graph systematically integrates and displays the knowledge framework within the field of traditional Chinese medicine (TCM). This not only contributes to a deeper comprehension of traditional Chinese medical theories but also provides robust support for the intelligent decision systems and medical applications of TCM. Against this backdrop, this paper aims to systematically review the current status and development trends of TCM knowledge graphs, offering theoretical and technical foundations to facilitate the inheritance, innovation, and integrated development of TCM. Firstly, we introduce the relevant concepts and research status of TCM knowledge graphs. Secondly, we conduct an in-depth analysis of the challenges and trends faced by key technologies in TCM knowledge graph construction, such as knowledge representation, extraction, fusion, and reasoning, and classifies typical knowledge graphs in various subfields of TCM. Next, we comprehensively outline the current medical applications of TCM knowledge graphs in areas such as information retrieval, diagnosis, question answering, recommendation, and knowledge mining. Finally, the current research status and future directions of TCM knowledge graphs are concluded and discussed. We believe this paper contributes to a deeper understanding of the research dynamics in TCM knowledge graphs and provides essential references for scholars in related fields.Keywords
As a millennia-old medical system in China, traditional Chinese medicine (TCM) plays a vital and irreplaceable role in maintaining public health and treating diseases. This is attributed to its unique theoretical framework, extensive practical experience, and notable therapeutic effects [1]. Over time, TCM has undergone continuous innovation, showcasing its distinct advantages not only in disease treatment but also in health preservation and disease prevention. Its global recognition and attention have been steadily increasing [2].
With the widespread application of information technology in TCM, a substantial amount of TCM-related data has accumulated, spurring a wave of data-driven research in the field [3]. However, the knowledge distribution within TCM is relatively decentralized, encompassing large amounts of tacit and relational knowledge. In this context, knowledge graph technology, which can effectively correlate, present, and apply such knowledge, holds significant potential and value for TCM. Consequently, researchers in this field have shown growing interest in the possibilities offered by knowledge graphs. Initially proposed by Google, knowledge graphs serve as knowledge bases to enhance search functionalities by describing real-world entities and their interrelations. Each knowledge graph comprises a vast number of triplet facts (
With advancements in artificial intelligence, knowledge graphs have become a key technology widely applied in various aspects of TCM, such as information retrieval, diagnosis, question answering, recommendation systems, and knowledge mining [5]. By establishing relational networks, knowledge graphs not only systematize TCM data management but also provide researchers with comprehensive analytical tools. This novel approach is anticipated to open new avenues for TCM development and accelerate knowledge accumulation and dissemination.
At their core, knowledge graphs are semantic knowledge repositories used to describe entities and their relations. These graphs consist of fundamental elements such as entities, relations, and attributes. Entities are connected through relations, forming a large network that visually and accurately represents associations between concepts and entities [4]. Based on their scope, knowledge graphs can be categorized as either general or domain-specific. While general knowledge graphs are relatively mature, domain-specific knowledge graphs, particularly in TCM, are still developing. Although general knowledge graphs provide broad coverage, they often fail to capture the intricate knowledge specific to specialized domains. In TCM, where entities and relations are complex and numerous, constructing domain-specific knowledge graphs is essential for tasks such as TCM information processing, semantic retrieval, and question answering.
Fig. 1 illustrates a simple knowledge graph representing the prescription “Gegen Qinlian Decoction.” TCM knowledge graphs typically employ triplets as their fundamental structure, with an “entity-relation-entity” or “entity-attribute-attribute value” format. Entities—such as medicinal prescriptions or diseases—are the foundational components of the graph [6]. Various relations, including ingredients, treatments, and compositions, can exist between entities. Attributes define characteristics such as medication usage or dosage, while the attribute value provides specific details, such as a drug’s content (e.g., “Liang,” a traditional Chinese unit of weight). Each triplet captures a factual relationship [7]. For example, the statement “Gegen Qinlian Decoction” has aliases like “Puerariae Decoction” and “Gegen Huangqin Decoction,” and its components include “lobed kudzuvine root,” “licorice,” “Baikal skullcap root,” and “Chinese goldthread rhizome.” It is used to treat conditions such as “myocarditis,” “diarrhea,” and “gastrointestinal syndrome.
Figure 1: Example of triples and TCM knowledge graph
Overall, the TCM Knowledge Graph has the following three key advantages:
• Comprehensiveness & systematization: TCM knowledge graph integrates the rich and complex knowledge of TCM, including classical literature, clinical practice, and case studies. Through structured presentation, it clearly demonstrates the systematic framework and key concepts of TCM, offering valuable references for research, teaching, and clinical practice.
• Relevance & depth: TCM knowledge graph reveals intrinsic connections between TCM concepts and uncovers the deep logic and interdependencies within the knowledge through correlation. By deeply mining the graph’s nodes and edges, it facilitates a comprehensive understanding of TCM.
• Shareability & inheritance: By organizing TCM knowledge in a structured, machine-readable format, TCM knowledge graph not only supports knowledge sharing but also ensures its accurate preservation, which addresses the fragmentation, loss, and inconsistent understanding that have traditionally plagued TCM due to oral transmission.
With the continuous advancement of technology, multimodal knowledge graphs have developed in the integration of information from various sources [8]. By merging data from different modalities, these graphs fully leverage their respective strengths, achieving a complementary effect. In TCM, the accumulation of multimodal information continues to grow, with medical devices capable of collecting data in various formats, such as audio, video, and images. Constructing multimodal knowledge graphs contributes to more reliable outcomes, offering comprehensive support for clinical decision-making in TCM [9]. Although there have been attempts to apply multimodal data in the TCM field, such as Li et al.’s multimodal knowledge graph for the Compendium of Materia Medica [10], research on large-scale multimodal knowledge graphs in TCM remains limited. Significant challenges remain, and further in-depth exploration is needed in this area. Additionally, interest in the automatic construction of knowledge graphs has been growing. For example, Weng et al. [11] proposed a framework for automatically building medical knowledge graphs based on semantic analysis. However, due to the complexity of TCM semantics, the fault tolerance of such graphs is low, making manual construction a crucial component in this field. In summary, for manual construction of single-modal knowledge graphs is an urgent component to be generalized in the TCM domain, which is also the meaning of the existence of this review.
We conducted a comprehensive search across several academic databases, including Google Scholar, PubMed, IEEE Xplore, and Web of Science. The search keywords used included, but were not limited to, “Traditional Chinese Medicine,” “knowledge graph,” and “TCM.” Our investigation revealed that the most recent review articles on TCM knowledge graphs date back to 2017 (according to Yu et al.’s work [5]). This highlights a common issue in TCM knowledge graph reviews, where updates are lagging, and the latest research findings and technological advancements have not been integrated in a timely manner, resulting in outdated information. Additionally, due to the interdisciplinary nature of TCM knowledge graphs, existing review literature often focuses on specific aspects, such as data construction, representation learning, or application scenarios. This has led to a lack of comprehensive coverage across the entire process from graph construction to application. Furthermore, there is a noticeable absence of review literature that classifies and organizes existing TCM knowledge graphs by subdomains, which impedes detailed literature research for scholars in various TCM fields.
To fill the gap, this paper provides a comprehensive and systematic review of TCM knowledge graphs. We summarize and evaluate the latest literature and advancements while looking ahead to future developments in the field. The construction process of TCM knowledge graphs is systematically organized, and we categorize and enumerate knowledge graphs within various TCM subdomains. Additionally, we introduce downstream medical application tasks based on TCM knowledge graphs and outline the current challenges and potential future directions in this domain.
In summary, our key contributions are as follows:
• To the best of our knowledge, this is the first review to categorize the existing literature on TCM knowledge graphs according to subfields within TCM. Moreover, we provide a comprehensive review of the current research landscape, from upstream knowledge graph construction to downstream applications.
• Our research shows that the latest English-language review literature on TCM knowledge graphs has not been updated since 2017 [5]. Therefore, this work serves as a critical update of the review literature in this area.
• We identify and discuss the challenges currently faced in TCM knowledge graph research and offer insights into future research directions, which we believe will become mainstream in the coming years.
The structure of this paper is outlined in Fig. 2. In Section 2, we provide a detailed introduction to the upstream technologies involved in constructing TCM knowledge graphs. In Section 3, we classify existing TCM knowledge graphs according to subdomains within the TCM field. Section 4 introduces downstream tasks based on TCM knowledge graphs, while Section 5 discusses the current challenges and explores future directions in the field. Finally, Section 6 offers a summary of this paper.
Figure 2: Framework of the systematic review
The construction of TCM knowledge graph is a complex task that demands comprehensive and technically demanding efforts, involving the comprehensive application of multiple knowledge graph construction techniques. This review combines the characteristics of the field of TCM and elaborates in detail on the technologies involved in constructing TCM knowledge graph. Presently, the pivotal technologies for TCM knowledge graph encompass knowledge representation, knowledge extraction, knowledge fusion, and knowledge reasoning [12]. The knowledge representation technology is dedicated to expressing the knowledge graph in a computationally processable format. Knowledge extraction technology aims to distill essential knowledge elements from diverse sources of TCM data. Concurrently, knowledge fusion technology is committed to integrating and resolving ambiguities in TCM knowledge, thereby establishing a semantically consistent and high-quality knowledge base for TCM. Additionally, knowledge reasoning technology enables the deduction of implicit knowledge. Fig. 3 illustrates the architectural diagram of the TCM knowledge graph system, elucidating the relations among the key technologies. In the contemporary landscape of TCM information processing, the comprehensive utilization of these technologies is paramount for the construction of a comprehensive and efficient TCM knowledge graph.
Figure 3: TCM knowledge graph construction architecture and downstream applications
2.1 TCM Knowledge Representation
Knowledge representation refers to expressing knowledge about the objective world in a format that computers can directly process, facilitating machine understanding and processing, which is of great significance for the construction, reasoning, and application of knowledge graphs [13]. In the field of TCM, this process encompasses a vast amount of empirical knowledge and inferential rules, such as the compatibility taboos of Chinese medicinal materials and the principles of prescription formulation. These aspects can be formalized through techniques like logical rules and path mining, thereby establishing reasoning relationships within the knowledge graph. The significance of TCM knowledge representation lies in how to employ appropriate methods to represent all facets of knowledge in Chinese medicine, facilitating machine understanding and processing. This is of great importance for the advancement of TCM in the realm of knowledge graphs [14]. With the development of technology, the knowledge representation of TCM faces numerous challenges as follows:
• Ontology technology requires transparent and standardized formal expressions. However, the diversity and non-standardization of TCM concepts make the construction of ontologies more complicated, greatly limiting their application in the field of TCM. For example, there are more fuzzy and cross-cutting contents in TCM concepts, such as inconsistent categorization of etiology, symptoms and therapies, which makes it difficult to accurately define and represent them when constructing ontologies.
• There are many confusing concepts in TCM. For example, in the description of symptoms, “limb trembling” and “generalized trembling” have different clinical significance, although they are similar. In knowledge representation, the definition and expression of entities are prone to ambiguity, leading to a decrease in the accuracy and operability of knowledge graphs. In the face of this challenge, it is often difficult for existing knowledge graphs to provide precise conceptual distinctions.
• Currently, most knowledge representation learning in the TCM domain relies on general-purpose domain models. However, due to the uniqueness and complexity of TCM data, existing models often fail to adequately capture the expertise and characteristics of the TCM domain. To date, there is no novel knowledge representation model specifically designed for the TCM domain, which limits the application of knowledge graphs in the TCM domain.
2.1.1 Ontology-Based Representation Method
In the realm of knowledge engineering, the early representational methods, such as first-order predicate logic and production representation, laid the groundwork for artificial intelligence research and applications. However, they exhibit inherent limitations in the representation, transmission, and sharing of knowledge. First-order predicate logic, despite its rigorous formalism, struggles with the complexities of relational dynamics and temporal variations. Meanwhile, production representation, widely employed in expert systems and rule engines, falls short in encoding semantic connections and hierarchical structures within knowledge domains. To address these limitations, the concept of knowledge ontology has been introduced [15]. As a structured framework for knowledge representation, ontology provides a formalized vocabulary for delineating conceptual relations and supports semantic querying and reasoning [16]. The application of ontology enables the precise organization and representation of knowledge, enhancing the efficiency of its transmission and sharing across diverse systems and user communities. Furthermore, ontology facilitates interoperability among disparate knowledge sources, establishing a robust foundation for knowledge integration and reuse. The integration of knowledge ontology significantly advances the capabilities of knowledge engineering in the representation and manipulation of knowledge, offering a powerful toolset for the construction of knowledge graphs [17].
Fig. 4 illustrates the close relation between the knowledge graph and ontology, where the knowledge graph enriches and expands based on the ontology, adding information at the entity level. Ontology places greater emphasis on describing the relation between concepts while emphasizing concepts. The construction of a knowledge graph can provide a more accurate reflection of ontology relations, while the construction of an ontology can establishes a more solid content foundation for the knowledge graph [18]. Knowledge graph ontology model consists of a pattern layer and an entity layer. Building the pattern layer requires pre-organizing the data structure, and the pattern layer regulates the data type through the ontology, so it is also called the ontology layer. Ontology is an abstract concept template. For example, defining an ontology triple (medicine, treat, disease), which stipulates that the fact objects can only be “medicine” and “diseases”, and the relation is “treat”. Instances describe things that exist in the objective world, often without subordinate objects, such as “Gegen”. Multiple ontology triples constitute the pattern layer, which is used to construct the logical structure of the knowledge graph.
Figure 4: Relation diagram of TCM ontology and knowledge graph
The construction of ontology primarily involves three methods: automatic construction, semi-automatic construction, and manual construction. TCM domain ontology usually invites domain experts to participate in manual construction and the seven-step method is a commonly employed semi-automatic construction approach in ontology modeling [19]. Due to the uniqueness and standardization of knowledge in the field of TCM, TCM knowledge graphs are modeled by mainstream ontology languages such as RDF (Resource Description Framework) and OWL (Web Ontology Language) [20]. A top-level design is created through the guidance of experts and then the data extraction and filling are carried out to complete the construction of the instance layer. In this way, the structure of the top-down knowledge graph is generated. At present, some ontologies of knowledge graphs in the field of TCM are sharable and reusable because of their accurate and standardized expression concepts.
Using ontology to establish knowledge models has become a common method for TCM knowledge analysis, and it is also a main direction of TCM knowledge representation research. In ontology representation, class-based and instance-based ontology representation methods have their own focuses, and both of them together constitute the core structure of knowledge graph. The class-based ontology is mainly used to define the conceptual hierarchy of knowledge graph, and organize the knowledge in the domain through abstract concepts and categories [21]. For example, in the knowledge graph of TCM, concepts such as herbs, diseases, symptoms, etc. can be represented by classes, and their subordination and association can be described by the hierarchical relationship between classes. Instance-based ontology representation, on the other hand, focuses on the representation of concrete entities, with each instance representing an actual object or event in the knowledge graph [22]. For example, a specific Chinese herbal medicine (e.g., “Angelica sinensis”) or a certain disease (e.g., “liver depression”) can be represented as an instance. Instances usually inherit class attributes and relate to other instances or classes to form a richer knowledge network. Yu et al. [5] constructed an ontology with TCM health as the core description object, providing a relatively complete framework for the construction of TCM health knowledge graphs. Zhu et al. [23] constructed ontology in the asthma domain and achieved knowledge sharing and reasoning. Cui et al. [24] created the TCM ontology of acne, providing knowledge support for clinical decision-making and other applications. However, ontology requires a clear and standardized formal representation. Due to the complexity and diversity of concepts within TCM, ontology construction for the field is more intricate, and its potential applications are constrained [25]. Despite the difficulties, establishing a clear and standardized expression of TCM knowledge holds immense value for the purposes of knowledge sharing and inheritance within the field.
2.1.2 Knowledge Representation Learning
Most traditional methods of representing knowledge can only capture explicit and discrete knowledge [26]. Representation learning techniques have garnered widespread attention. It refers to expressing the semantic information of the research object into dense low-dimensional vectors through deep learning methods which can solve the problem of sparse data as well as efficiently calculate the complex semantic relations between entities and relations. By enhancing the translation model TransE [27], numerous models for knowledge representation learning incorporating complex relation modeling have been proposed, such as TransH [28], TransD [29], TransR [30], SHGNet [31], TEGS [32], etc. Meanwhile, an increasing number of alternative models have been proposed, such as DeepWalk [33], node2vec [34], and LINE [35], etc. However, the knowledge representation learning method in the field of TCM is still in the exploring stage. Zheng [36] addressed the issue of the inadequacy of existing translation models in effectively representing TCM knowledge. To overcome this, he incorporated Chinese features such as pinyin, strokes, and structural information and encoded them, and then employed a translation model for triple representation learning, mapping entities and relations to a vector space. Wang et al. [37] proposed a new TCM entity representation learning method TCME based on heterogeneous information graphs by constructing TCM entity vector representations by aggregating diverse neighbor nodes on various edges. Jin et al. [38] utilized graph convolutional networks to acquire symptom embeddings and herbal embeddings concurrently from various graphs of symptom-herb, symptom-symptom, and herb-herb. This approach allowed them to create a more comprehensive representation of symptoms and herbs. Yang et al. [39] constructed a medicine knowledge graph, introduced medicine attributes as additional auxiliary information, and proposed a graph convolution model with multi-layer information fusion to obtain symptom and medicine feature representations with affluent details and less noise.
Knowledge representation is a focal point of research within the domain of knowledge graphs. Existing studies have corroborated that through the lens of knowledge representation learning, the ability of computers to comprehend and process medical text, such as TCM prescriptions, can be significantly enhanced by mapping the terms or sentences from the text to nodes or edges within a knowledge graph. This facilitates the computational understanding and manipulation of knowledge contained within medical texts. Nonetheless, the realm of knowledge representation faces certain challenges. For instance, there is a need to represent the intricate relations and conceptual hierarchies inherent in TCM, to grapple with the ambiguity and uncertainty present in medical texts, and to enable automated reasoning regarding TCM knowledge. Despite these challenges, the ongoing advancements in artificial intelligence and natural language processing hold promise for substantial growth and progress in the field of knowledge representation.
In addition, as a form of knowledge representation learning, embedding methods are not only used for vector representations of entities and relations during knowledge graph construction, but also often as downstream applications for classification and inference [5]. In the Section 5, we will see how these embedding methods are applied to TCM downstream applications. In these application scenarios, the vector representations obtained through knowledge representation learning will help to improve the accuracy and efficiency of the system, thus better serving the automation and intelligence needs of the TCM domain.
TCM knowledge extraction refers to the automated process of identifying and extracting entities such as drugs, symptoms, and diseases, as well as the relations between them, from TCM textual data. Typically, the knowledge extraction process comprises several sub-tasks, including entity recognition, relation extraction, and attribute identification. Generally, TCM knowledge extraction involves extracting information related to TCM from TCM texts, such as the names of medications, symptom names, and their interrelations. However, there are still significant challenges in the extraction process of TCM, which are outlined as follows:
• Although TCM terminology has been standardized to some extent, a strict naming system has not yet been formed. This has led to the existence of many homophones, remote words and polysemous words in practical applications, which can easily lead to confusion and ambiguity. For example, “qi” can have different interpretations in different contexts, adding to the complexity of automatic processing.
• Entity naming in TCM tends to be more complex, often containing sub-entities or nested entities. This is particularly evident in the naming of Chinese medicine formulas. For example, “Guizhi Fuling Pill” is a traditional Chinese medicine prescription, in which “Guizhi” and “Fuling” are TCM words. When representing these complex naming structures in a knowledge graph, it is a challenging problem to accurately distinguish and represent the subentities.
• Currently, there is a lack of large-scale and standardized annotated corpus in the field of TCM. Due to the lack of high-quality TCM training datasets, the performance of existing models in the TCM domain is limited. In order to improve the accuracy of the models, applicable training sets need to be constructed manually, while the high-quality labeling is still mainly done manually, which is not only time-consuming and labor-intensive, but also puts higher demands on experts in the field of TCM.
TCM entity extraction refers to the process of identifying and classifying specific entities within TCM textual data, which include but are not limited to ingredients of traditional Chinese herbs, disease names, symptom descriptions, and treatment methods. This process involves locating relevant words or phrases from the original text and accurately labeling them with predefined category tags. Known as Named Entity Recognition (NER), entity extraction is a crucial branch of information extraction. As shown in Fig. 5, the advancement of entity extraction technology in TCM research has evolved from initial rule-based methods to the current deep learning models. This series of technological advancements, as illustrated in the figure, not only enhances the accuracy of recognizing TCM-specific entities such as herbs, diseases, and symptoms but also promotes the automation and intelligent processing of TCM information.
Figure 5: Development of TCM entity extraction models
Rule-based methods involve constructing a large number of rules by experts to infer possible named entities. Friedman et al. [40] developed the earliest MedLEE (Medical language extraction and encoding) system, which identified disease names and disease modifiers in X-ray reports based on lexical and syntactic rules, and normalizes the disease names and maps them to the Medical Entities Dictionary (MED) to encode the diseases. Disease names are normalized and mapped to the MED to encode diseases. Dictionary-based methods require the establishment of a named entity dictionary, and named entities are identified by matching queries against the dictionary or a domain-specific knowledge base. Although high accuracy rate, it relies entirely on rules and dictionaries constructed by experts. Wang et al. [41] referred to the symptom name dictionary. They matched symptom names from the segmented clauses according to the maximum matching algorithm, identifying most of the symptom names with greater accuracy included in the symptom name dictionary. Although rule-based and dictionary-based methods have some drawbacks, they are generally used in conjunction with other methods. The addition of rules and dictionaries to subsequent statistical learning and deep learning approaches can enhance accuracy.
Subsequently, numerous studies applied statistical machine learning methods to extract TCM entities. The statistical learning-based methods mainly utilize manually annotated corpora for training, from which relevant features and parameters are statistically computed to establish recognition models. The common models currently used are Conditional Random Field (CRF) model, Hidden Markov Model (HMM), Maximum Entropy Markov Model (MEMM), and Support Vector Machine (SVM). An increasing number of studies have demonstrated the effectiveness of CRF models in the field of TCM, and it gradually becomes the main model for TCM entity recognition [42]. Nevertheless, CRF has a strong dependence on feature selection. It takes a long time to train the model when the training corpus is extensive.
Some studies have combined multiple methods for named entity recognition in practical applications. The combination of rule-based and statistical-based methods has become a trend, where statistical-based methods can process the results of rule-based methods as features, thus leveraging the advantages of both methods. Liu et al. [43] used a rule-based approach to assist the construction of TCM clinical medical record datasets. They used CRF to conduct name extraction experiments, and compared it with HMM and MEMM. The results show that CRF can make full use of the features of cases in TCM and has a better performance in entity recognition. Wang et al. [44] employed rule-based techniques to cleanse the medical case corpus during pre-processing, followed by the application of CRF for identification and labeling. Therefore, a combination of rules and CRF was utilized for named entity recognition research. Li et al. [45] combined CRF with rules to initially identify medical record entities, and optimized the results based on rules. Liang et al. [46] used SVM to filter out sentences that did not contain medicines vocabulary in order to remove unreasonable data from the dataset and enhance the performance of CRF. Statistical-based methods have led to a significant improvement in performance, but they also necessitate manual annotation by individuals possessing professional domain expertise.
Deep learning has become a research hotspot in machine learning in recent years. Deep learning-based methods enable machines to learn potential feature templates automatically. Yuan et al. [47] generated different word vector features by using two methods of constructing vectors, word2vec, and node2vec. Then the methods based on CRF and SVM were subjected to ten-fold cross-validation, which demonstrated that the named entity extraction algorithm with deep representation outperforms the traditional non-deep representation named entity recognition algorithm. Some studies constructed NER models based on Bidirectional Long and Short-Term Memory networks (BiLSTM) and CRF to improve the accuracy of TCM entity recognition [48]. The model structure is shown in Fig. 6.
Figure 6: BiLSTM-CRF model structure based on Chinese characters
Gao et al. [49] utilized the BiLSTM with a peephole mechanism to enhance the performance of TCM NER. This method effectively identified named entities for symptoms in TCM medical records and improved the recall ability of the conventional CRF model. Zheng et al. [50] used the TCM term recognition model BERT-BiLSTM-CRF and achieved excellent performance in TCM term recognition task. Zhang et al. [51] proposed a semi-supervised embedded Semi-BERT-BiLSTM-CRF model. It considered the characteristics of TCM classics and selected features from cleaned text information. Following this, a BERT-BiLSTM-CRF model was trained using a limited amount of labeled data. Jia et al. [52] proposed a span-level NER TCM entity extraction model based on distant supervision to obtain domain dictionaries from TCM knowledge graphs, using BERT pre-trained language models as text feature encoders, and using multi-layer neural networks to detect and classify entities. Jin et al. [53] introduced a knowledge attention vector model to implement an attention mechanism between the hidden vector of the neural network and the knowledge graph candidate vector, which takes into account the influence of the previous work. Cheng et al. [54] incorporated dictionary features into a multi-task neural network to enhance the recognition of rare entities to improve the performance of NER main task.
After analyzing and summarizing the aforementioned four methods, their respective advantages and disadvantages are compiled and presented in Table 1.
Relation extraction refers to the extraction of relations between entities from the text containing multiple entities [55]. TCM relation extraction aims to identify and extract semantic relations between TCM entities, as shown in Fig. 7. Initially, relation extraction relied on a rule-based approach. Fang et al. [56] applied a rule-based method to sentences to extract relations between herbal medicines, genes, and diseases. Yu et al. [57] proposed a method of matching ontology-generated templates with text information to automatically identify new entity relations within the text, thereby constructing a TCM text information extraction system. Machine learning methods were then introduced into the field and achieved good results. Yang et al. [58] constructed a support vector machine classification model for relation extraction after conducting feature extraction. The model was used to identify the relation between TCM prescriptions and diseases. However, classic machine learning algorithms primarily improve the accuracy of relation extraction through complex manual feature engineering. Thus, it limits the potential of classical machine learning to improve accuracy.
Figure 7: The general framework of the TCM relation extraction system
In contrast, deep learning methods can eliminate the need for feature engineering. Luo et al. [59] proposed a relation recognition algorithm based on a bidirectional long short-term memory network that integrates a gradient boosting tree, so as to improve the effect of relation recognition. Wan et al. [60] put forth a novel heterogeneous network-based relation extraction model, which differs from previous approaches that focus on extracting individual relations from a single sentence or document. This model has the capability to extract multiple types of relations across the entire corpus. Bai et al. [61] designed a convolutional neural network with a segmented attention mechanism, which can extract local semantic features through word embedding, and then classify relations by concatenating different embedding features. Zheng et al. [50] constructed a multi-label and multi-classification task model for recognition relations and identified multiple relations. Han et al. [62] proposed the PCNN with Attention model to extract the joint relation after completing the entity extraction task.
For TCM entities, attribute extraction refers to constructing a list of attributes for TCM entities, for example, the attributes of “Poria” are “ distribution, dosage, toxicity”, and so on. The attributes of an entity can be regarded as a relation between the entity and the values of the attributes. Therefore, the problem of attribute extraction can be transformed into a problem of relation extraction.
After the entities, relations, and attributes are obtained through knowledge extraction, it’s possible that there is a significant amount of information that is either unnecessary or inaccurate. So further fusion is required [63]. Knowledge fusion is a critical stage in the creation of a knowledge graph, as it enables the merging of diverse, disparate sources of data and triple information extracted from knowledge extraction into a unified framework. This integration serves to eliminate ambiguity and redundancy in the data, leading to an enhancement in the overall quality of the resulting knowledge graph. Integration of TCM knowledge still presents a set of challenges due to the unique characteristics of this field:
• The information sources of TCM are extensive and complex, with overlapping data and fuzzy relationships. Many knowledge fusion techniques in generalized domains are difficult to be directly migrated and applied to the TCM domain. For example, when dealing with various types of information such as herbal medicines, prescriptions, symptoms, etc., the vagueness and uncertainty of the relationships often lead to poor knowledge fusion and fail to fully demonstrate the complex knowledge structure of the TCM domain.
• When merging TCM ontologies, different ontologies may describe the same type of entities, resulting in entity alignment difficulties. Currently, the alignment problem of TCM ontologies has not been effectively solved. This not only increases the difficulty of knowledge integration, but also hinders the interoperability of cross-domain knowledge, making ontology fusion in the TCM domain relatively challenging [64].
• When performing entity alignment, most TCM databases lack a priori data, which means that experts need to manually label the data when constructing the training data. Manual labeling is not only time-consuming and labor-intensive, but due to the complexity of the TCM domain, the quality of the labeling greatly depends on the knowledge and experience of the domain experts, which makes constructing high-quality training sets a difficult task.
Ontology fusion integrates and unifies ontologies from two or more different domains or systems. The goal is to eliminate heterogeneity between different ontologies and build a unified, ontology-level body of knowledge. The main challenges of ontology integration include conceptual tautology, hierarchical inconsistencies, and alignment of attributes and relationships [65]. When Miao et al. [66] performed ontology fusion, they kept only one of the multiple names of the same entity, and aligned diseases, symptoms, and their attribute entities from various knowledge bases. However, due to the complexity of TCM ontology, there are still difficulties in the integration of TCM ontology.
Instance fusion mainly refers to the matching and merging of different instances representing the same entity in different knowledge bases or knowledge graphs. Instance fusion usually involves processes such as data cleansing, entity identification, and elimination of duplicates to ensure that the same entities are consistent after fusion [65]. The key technologies of the instance fusion in TCM mainly include entity alignment and entity disambiguation. Entity alignment is also called “coreference resolution”, “entity matching”, etc., and refers to the situation where multiple descriptions correspond to the same entity object. Entity alignment is a well-studied knowledge fusion task in TCM knowledge graphs. A TCM entity usually has many different expressions. For example, the aliases of “Danggui” include “Gangui”, “Yungui”, “Maweigui” and so on. Most entity alignment methods in TCM are based on rules and shallow machine learning methods, which cannot utilize the deeper semantic information of entities. Guo [67] primarily depended on the label of experts in TCM and the naming of relevant norms to achieve the purpose of entity alignment. Zhang [68] employed entity attribute relation similarity to align certain entities and text similarity to align others. Due to the inconsistent use of words in TCM, knowledge fusion is not effective after using similarity calculation, and domain experts must conduct a manual review. With the development of deep learning, Luo et al. [69] used RoBERTa as the pre-training model, BiLSTM as the feature extractor, and CRF as the sequence annotation. They manually annotated the corpus to recognize six entity types: symptoms, tongue diagnosis, pulse diagnosis, prescriptions, syndrome differentiation, and traditional Chinese medicine.
Compared with entity alignment, there are fewer studies on entity disambiguation, which is also an instance-level fusion task. Entity disambiguation is mainly aimed at resolving the problem of polysemy. Zhang et al. [70] disambiguated the teacher-inheritance relation, user experience, knowledge, etc., by professionals. Since mechanical participles can be wrong, for example, “gan mao(cold)” is a symptom, but “gan mao ling(cold remedy)” is a medicine, context-based disambiguation is required. Zhang et al. [71] introduced BERT to the entity extraction task, considered the context information, made full use of the global information, and had a great advantage in entity disambiguation.
The available public resources for TCM knowledge graphs are scarce and of relatively low volume, resulting in limited and shallow applications. Furthermore, knowledge fusion at the knowledge graph level is a rarity. Yang [72] constructed a COVID-19 scientific literature knowledge graph, a western medicine treatment knowledge graph, and a TCM treatment knowledge graph. The TCM treatment knowledge graph and the western medicine treatment knowledge graph are regarded as subcategories of the “drug therapy” knowledge category so as to realize the fusion of knowledge graph levels.
Knowledge fusion technology in TCM has developed to a certain extent, but it still requires a lot of manual intervention at this stage. There is ample opportunity to enhance the quality of knowledge fusion in this field, and there is still a need for the development of fusion algorithms tailored to its unique characteristics.
The main process of knowledge reasoning involves modeling the features and learning parameters of existing entities and relations in a knowledge graph to mine or infer unknown or implicit new knowledge. In the TCM knowledge graph, knowledge reasoning can help doctors diagnose diseases and provide treatment options [73]. Currently, TCM knowledge reasoning still faces difficulties such as follows:
• Knowledge reasoning in TCM is limited by the lack of classical and high-quality data samples. In the field of TCM, data errors occur from time to time and the error tolerance rate is extremely low, thus, it is necessary to rely on the participation of TCM experts in the data labeling process to ensure the accuracy and reliability of the data. This high demand for expert dependence increases the difficulty of knowledge reasoning.
• Most of the existing TCM knowledge reasoning methods only consider the structured information of the triad in the knowledge graph, and ignore the importance of the four diagnostic methods of observation, smelling, questioning and cutting in the TCM diagnosis and treatment process. The credibility of the knowledge reasoning in TCM relying solely on text or image information is low, and it is difficult to truly reflect the TCM diagnosis and treatment process. Therefore, there is an urgent need to further enrich the multimodal information in the field of TCM to improve the accuracy and reliability of knowledge reasoning.
• TCM has undergone a long history of development, but the effective utilization of its knowledge is often hindered by time and space constraints. The accuracy of current TCM knowledge reasoning techniques still need to be improved when dealing with cross-era or cross-geographical TCM knowledge. Therefore, how to optimize the reasoning process of TCM knowledge in time and space dimensions has become an urgent problem in current research.
According to the knowledge reasoning used in TCM knowledge graphs, it can be divided into logic rule-based reasoning, distributed representation-based reasoning, and neural network-based reasoning [74].
2.4.1 Logic Rule-Based Reasoning
The logic rule-based reasoning approach is mainly inspired by traditional knowledge inference methods such as rule-based reasoning or logical reasoning. It uses simple rules or statistical features to perform inference on knowledge graphs. Xie et al. [75] used Jena to apply rules to knowledge graphs for knowledge reasoning, and generated some new relations, which proved the feasibility of rule-based reasoning. Wang et al. [76] proposed a diagnostic generation model for knowledge graph enhanced transformer (KGET) networks. At the same time, by introducing knowledge from the medical field, the KGET for CM diagnosis generation model was established. By introducing knowledge from the medical field, the reasoning ability of diagnosis was enhanced. Long et al. [77] proposed a diagnostic and treatment system for common psoriasis based on case-based reasoning and approximate reasoning using fuzzy logic. Yang et al. [78] proposed a TCM model based on the temporal knowledge graph, which can effectively represent the dynamic changes of clinical knowledge over time. Three temporal inference rules were established to fulfill the temporal intent of a search sentence, which are based on the logical correlation among the start time, end time, and duration. When reasoning at the ontology concept level, the rule language Semantic Web Rule Language (SWRL) can add custom rules to the ontology model. Fu et al. [79] proposed an association rule mining (AMIE) based on the incomplete knowledge base and a random walk diagnosis and treatment inference algorithm to address the standardization of clinical diagnosis and treatment for acute abdomen and the inheritance of experience in traditional Chinese medicine. By recommending personalized diagnosis and treatment plans for cases, they provided information services and technical support for grassroots doctors. Bao [80] used SWRL rule language and Jess reasoning engine to implement diagnostic reasoning and prescription recommendation.
2.4.2 Distributed Representation-Based Reasoning
The distributed representation-based reasoning approach primarily involves acquiring a low-dimensional embedded representation of entities and relations and conducting subsequent medical reasoning using this semantic representation. This approach effectively utilizes information from the knowledge graph but cannot incorporate prior experience during the modeling phase. The main models are tensor decomposition, distance model, and semantic matching model. Zheng [36] decomposed knowledge reasoning into link prediction tasks between multi-layer entities of knowledge graphs based on chronic kidney disease TCM medical records. Based on knowledge graph representation learning for chronic kidney disease, the feature extraction of path vectors composed of entities and relations in the knowledge graph is performed through a multi-dimensional convolutional neural network to realize the link prediction task.
2.4.3 Neural Network-Based Reasoning
The neural network-based reasoning methods utilize neural networks to learn from the triplets in the knowledge graph. Integrating CNN, RNN, GNN, and other models into representation learning or logical rule models establishes a predictive model using sequential paths between entities for relevant reasoning. The learning and generalization abilities are strong, and modeling triplets reduces the calculation difficulty. Xie et al. [81] introduced reinforcement learning algorithms based on TCM knowledge graph to excavate the hidden relations between entities, obtaining inference paths, deduce paths from symptoms to symptoms, and obtaining all possibilities through the relation between symptoms and causes.
To enhance comprehension and facilitate comparisons among different reasoning methods, each method and their respective advantages and disadvantages are briefly described in Table 2.
Due to the rich knowledge in the field of TCM, there may be a large amount of identical or contradictory information, and the construction of a knowledge reasoning model is still complicated. TCM knowledge reasoning is gradually evolving to cope with more complex reasoning tasks. By leveraging the knowledge graph of TCM, knowledge reasoning can further enhance the semantic relations within the graph, which has emerged as a research focus in recent years. This approach can provide clinical knowledge or aid in medical diagnosis for healthcare professionals and patients.
TCM is a vast and intricate field, making it impractical to construct a comprehensive knowledge graph containing all information and data. Therefore, there is a need for a comprehensive categorization and assessment of the TCM knowledge graph for each sub-domain. Consequently, researchers often opt to build subdomain-specific knowledge graphs within the realm of TCM based on practical needs. This personalized construction approach aids in precisely meeting specific research objectives, enhancing the utility and operability of information. For instance, an expert studying the correlation between TCM and a particular disease may focus on the relation between symptoms and medications, while another may concentrate on the pharmacological effects of Chinese medicinal herbs. Such customized knowledge graphs facilitate in-depth exploration of specific domains, advancing research and application in TCM. In the process of constructing TCM knowledge graphs, considering practical needs contributes to the efficient integration and utilization of the abundant knowledge within the TCM field.
Currently, progress has been made in the construction of TCM knowledge graphs in subdomains such as fundamental TCM theories, clinical practices, and herbal formulations. Notable subdomains include acupuncture, ethnic medicine, and others. Through reviewing the scientific literature in the field of TCM over the past decade, we categorized the TCM knowledge map into seven major categories based on the types of data sources used to construct the knowledge map, namely, “Basic Theory”, “Prescription”, “Herb”, “Syndrome”, “Clinical”, “Health Regimen”, and “Others”. In total, there are seven major categories. Frankly speaking, it is labor-intensive and resource-intensive to fully and clearly classify all the subdomains of the TCM knowledge graph, so this part of work is also one of our research directions in the future. Table 3 clearly summarizes the TCM subdomain knowledge graphs and the data sources used to construct these graphs.
The knowledge graph of TCM has achieved significant progress and has been applied in various fields. Knowledge graphs can assist information understanding and improve retrieval accuracy, recommendation and question answering performance. The TCM knowledge graph can assist doctors in diagnosis and treatment and can help discover new knowledge from a large amount of data. Many studies have developed a knowledge service platform based on medical application. This paper summarizes the application of TCM knowledge graph as TCM information retrieval, TCM diagnosis, TCM question answering, TCM recommendation, and TCM knowledge mining.
Knowledge graph was originally proposed to optimize search engine retrieval. TCM information retrieval greatly improves the efficiency of inquiry and learning, providing a platform for the public to access information. Deng et al. [110] integrated a variety of TCM data resources and used a knowledge graph to clearly present the relation among diseases, syndromes, treatments, prescriptions, and medicines in TCM medical records. Compared with conventional knowledge retrieval, it can help users efficiently explore the knowledge derivatives they are interested in. Liu et al. [111] used ontology technology to construct a traditional Chinese medicine stroke ontology. Under the guidance of Stanford University’s Seven Step Method, they integrated and analyzed relevant information in the field of stroke, sorted out the basic knowledge framework of the disease, and used Protein 5.5.0 to construct a traditional Chinese medicine stroke ontology, completing knowledge representation, knowledge storage, and information retrieval in this field. Zuo et al. [85] showed Zhao Bingnan’s academic thought and knowledge system with the help of knowledge graph. It is possible to query the diseases that can be treated by the academic school of Zhao Bingnan, as well as the prescriptions used and another related knowledge.
In the process of TCM diagnosis, the level of TCM knowledge and clinical experience may affect the diagnostic proficiency of the doctor. The knowledge reasoning ability and knowledge discovery ability can solve complex problems. Doctors can use the knowledge graph to assist in obtaining recommended medication or diagnosis results, thereby helping TCM decision-making. Long et al. [77] relied on domain ontology, support rule-based knowledge reasoning, and provide users with clinical decision support for psoriasis TCM syndrome differentiation diagnosis. Ruan et al. [97] used the Drools engine and conversion program to automatically convert the data in the knowledge graph into inference rules that the inference engine can use, and combined with the patient's factual data to assist doctors in prescribing. In recent years, Zhang [68] constructed a tongue image diagnosis and treatment system based on knowledge graphs. The system simulates the TCM diagnostic process by diagnosing symptoms based on user symptoms and tongue status, and provides treatment plans accordingly. Sun et al. [103] used the rheumatoid arthritis knowledge graph to provide doctors with diagnoses and treatment suggestions based on the symptoms, diseases, examinations, and other information in the medical records. Wang et al. [76] proposed a pre-trained dialogue text generation model that integrates medical knowledge to complete the task of generating traditional Chinese medicine diagnostic text for lung cancer. This task utilizes the semantic information contained in the pre trained model and integrates knowledge from the domain knowledge base to improve the effectiveness of the model in generating traditional Chinese medicine diagnostic texts. Yang et al. [112] used the knowledge graph representation learning method to realize syndrome recommendations, and built a syndrome diagnosis and decision system based on TCM knowledge graphs. Li et al. [113] developed a knowledge-based recurrent neural network model utilizing the cerebral palsy knowledge graph to aid in the diagnosis of cerebral palsy.
The development of knowledge-based intelligent question answering is a current research focus in human-computer interaction. By utilizing knowledge graphs as a source of answers, these methods can more accurately understand natural language questions and provide more precise answers based on the user’s true intention. Currently, there are three main methods for knowledge-based question-answering: template matching, semantic parsing, and deep learning.
As shown in Fig. 8, the template matching approach is commonly used to parse natural language. in TCM field. Compared with the other two approaches, the template-based method only requires the design of question templates based on common questions and matches the templates through methods such as similarity calculation. It does not require learning on a large corpus of manually labeled language data, which is suitable for domain-specific question answering or high-frequency question answering. However, it also has disadvantages such as the need to establish a large template library and the high cost of manual annotation. Ruan et al. implemented three processes of word segmentation, template matching and template translation to realize the question and answer of TCM knowledge [97]. Wen et al. [114] designed an ontology-based automatic question-answering system for TCM coronary heart disease by constructing TCM Coronary heart disease ontologies and common questions and using various processing methods according to the characteristics of the questions. Jia et al. [105] transformed the natural language described by patients into standardized knowledge representations such as symptoms taking “menstrual disease” as an example. The associated knowledge in user questions is discovered based on the entities and relations in the knowledge graph. And through further reasoning, the answers to user questions are obtained using the weights of key concepts and entities. Yin et al. [115] designed a question template based on the common questions of hepatitis B patients using Word2Vec to calculate the text similarity between the question and the design template and completed the matching to realize the medical question and answer of hepatitis B disease. Gao et al. [116] first identify entities in the sentence and then generate a sentence vector by combining TF-IDF and word embeddings. They match the most similar question and answer template, search for answers in the knowledge graph based on the semantics of the template and the entities in the question, and finally answer the question based on the returned data. Zou et al. [117] used the open-source data of “Compendium of Materia Medica” as the data source to build a TCM knowledge graph and built a TCM question-answering system containing three categories of TCM knowledge and TCM syndromes.
Figure 8: Work flow chart of TCM question answering system
Unlike template-based methods, semantic parsing-based methods are less commonly used in the field of TCM. Luo [118] used GDBT-based question point recognition, performed sub-graph mapping, and finally delivered the final answer based on weight information. It makes up for the shortcoming of the narrow question range due to limited question templates and answers. With the development of technology, there have been some studies using deep learning for automatic question-answering in TCM. Deep learning-based methods do not require manual construction of template libraries, but currently, the processing of complex questions needs to be improved, and the training process can be lengthy. Due to the professional nature of problems in TCM, understanding the semantics of questions still needs to be strengthened. Liu et al. [99] used the deep learning model to analyze the question's intent in the question-answering module to understand the question. They provided ordinary users with an interface of single-round question-and-answer and multi-round dialogue. Lv [100] designed and implemented a question semantic graph representation model based on a gated graph neural network. By explicitly modeling the structure of semantic relations in the question, it is possible to uncover deeper semantic relations in the question, thus improving the performance of answering complex questions in question answering tasks. Zhou et al. [119] leveraged the powerful knowledge learning ability of state-of-the-art LLMs to address the efficient acquisition and utilization of TCM epidemic disease knowledge. They collected content related to epidemic diseases from 194 ancient TCM books, as well as the knowledge graph of TCM epidemic disease prevention and treatment, and built the large TCM epidemic disease model EpidemicCHAT.
Compared with traditional search engines, recommendation systems can consider users' personalized needs and predict their needs based on their preferences, habits, and other factors. The most suitable TCM information can be provided to users with the help of the TCM knowledge graph. Yu et al. [5] combined the knowledge graph with expert scoring, user click-through rate, and other factors. They recommended TCM health-preserving knowledge for users based on their personal health information. Zhao et al. [120] proposed a knowledge graph embedding-enhanced topic model. The hidden association between the co-occurrence information in TCM case records and the similarity of TCM entity vector representations is mined from two aspects, improving the performance in TCM recommendation tasks. Jin et al. [38] fed multiple symptom embeddings into a multi-layer perceptron and integrated them with herbal embeddings to complete herbal medicine recommendations. Qi et al. [121] combined large-scale language models with GNN to establish a novel Chinese medicine prescription recommendation model, improving the accuracy of prescription recommendation. Based on the co-occurrence characteristics of symptoms and traditional Chinese medicine, they constructed symptom maps, syndrome medicine maps, and traditional Chinese medicine maps. Using GCN, embeddings of symptoms and herbs were obtained, and then integrate symptom embeddings with insights from large language models, and integrate auxiliary information from external knowledge graphs into herbal embeddings. The final list of recommended herbs was generated through embedded interaction with symptoms and herbs. Qu et al. [122] proposed an intelligent recommendation method for Chinese medicine based on multimodal knowledge graph attention network. They construct a TCM multimodal knowledge graph containing rich semantic and contextual information by integrating heterogeneous data from different sources. Then, the graph attention network is used to enhance the feature representations of users and TCM entities, and these enhanced feature representations are finally used to achieve personalized recommendations.
In recent years, scholars have applied association rule discovery, cluster analysis, complex network analysis and other methods to the field of TCM, providing assistance in discovering the compatibility rules of herbal formulae, assisting in prescription formulation, and developing new drugs. Yan et al. [92] used the FP-Growth algorithm to analyze the prescription data and mine the cross-entity relation in the prescription network, which helps explore the core medication mechanism of TCM in treating different groups of people with the same disease. Xie et al. [87] built a personalized knowledge graph based on TCM basic theory. They used data mining to discover implicit and new knowledge and proposed a data-driven knowledge mapping method for personalized knowledge discovery. Gong et al. [123] proposed a knowledge graph relational path network for target prediction of TCM prescriptions. Semantic information and high-level structure of knowledge graphs are extracted by knowledge graph embedding and graph neural network. Emphasizing relations in knowledge graphs when aggregating statements improves the performance of predicting relations between prescriptions and targets. Yin et al. [124] proposed a new graph contrastive representation learning framework TCMCoRep with explicit syndrome awareness. For a given symptom set, the predictive representation of TCMCoRep can not only find high-quality prescriptions, but also, based on the diagnostic philosophy of traditional Chinese medicine in real life, generate prescriptions through syndrome perception, and clearly detect corresponding syndromes.
Knowledge graph provides a new way of storing and utilizing knowledge for the field of TCM, and has a wide range of application prospects. In this paper, based on introducing the development, construction, and application of TCM knowledge graphs, we analyzed the current research status of TCM knowledge graphs. Research on the TCM knowledge graph has made some achievements, but it still faces challenges, and there is still great potential for the future development of the TCM knowledge graph.
(1) Construction of TCM knowledge graph based on terminology standards [125]. Most of the data in TCM still exists in unstructured text such as documents, and ancient books. When constructing the TCM knowledge graph, it needs to be carried out under the guidance of the domain terminology standard. There is an urgent need to establish standardized terminology for symptoms, ancient medical terms, and other related areas [126]. Moreover, as TCM texts and encyclopedias are predominantly in Chinese, there are semantic ambiguity problems and information loss problems during the translation process of constructing and using the English TCM KG, making it difficult to maintain the original meaning of TCM terms in the translation process, which in turn affects the accuracy of the KG, and therefore a consistent English terminology standard in TCM is needed.
(2) The construction technology of TCM knowledge graphs still needs to be explored. Due to the professional characteristics of TCM and the absence of data sets with high quality, research on knowledge fusion and representation is still in its infancy. Although some studies have achieved good results on specific datasets, they typically do not have scalability and portability [75].
(3) Sharing of TCM knowledge graph. Despite the emergence of numerous knowledge graphs in the field of TCM, most of them suffer from limited dissemination, non-public content, and poor reusability. The construction process of a TCM knowledge graph may involve a considerable amount of redundant work. Additionally, many existing TCM knowledge graphs lack proper quality assessment after construction [111]. Therefore, the TCM knowledge graph supports sharing and collaboration, which can promote the development of the TCM knowledge graph [127].
(4) Multi-modal knowledge graph of TCM. Only relying on single-modal data of text or images cannot comprehensively cover the knowledge in the field of TCM. Most current TCM knowledge graphs focus on text data, and less attention is paid to images, videos, etc. Combining multi-modal information can extend the knowledge graph to provide a data basis closer to the actual scene for upper-level intelligent applications [128]. In addition, in the process of constructing the TCM KG, Chinese herbal medicines can be analyzed in more detail to extract key information such as their chemical composition and pharmacological factors, which can be used as an independent modality to construct a multimodal TCM KG. By integrating these multimodal data, it can not only enrich the expressive ability of the KG, but also provide more comprehensive data support for in-depth research in the field of pharmacology, and promote the application and development of TCM in pharmacological mechanisms, efficacy prediction, and drug development [129].
(5) The integration of large language models (LLM) with TCM knowledge graphs holds the potential to enhance the comprehensiveness and accuracy of TCM knowledge, addressing the fragmented nature of traditional knowledge representation [58]. Additionally, the automated analysis capabilities of large language models facilitate the efficient updating of knowledge graphs, ensuring their alignment with contemporary developments. The amalgamation of individualized healthcare data further promises to propel the development of personalized medical and therapeutic approaches, offering patients more precise diagnostic and treatment recommendations. This prospective outlook injects new vitality into the field of traditional Chinese medicine, fostering convergence with modern medicine and advancing both healthcare services and research standards.
In the coming years, the TCM knowledge graph will continue to be at the forefront of big data intelligence research and receive more attention. The knowledge graph can promote the intelligence of TCM and bring new development opportunities to the industry.
With the rapid development of technologies such as artificial intelligence, big data, and digital healthcare, the construction and application of knowledge graphs in the field of TCM are gradually gaining attention. This review systematically introduces the key technologies for constructing knowledge graphs in TCM and classifies existing instances of TCM knowledge graphs according to sub-domains within TCM. Furthermore, an analysis and discussion of downstream task scenarios based on TCM knowledge graphs are presented. In addition, we identify potential opportunities and outline promising future directions. This review fills the gap in the literature on knowledge graph reviews in the field of traditional Chinese medicine over the past five years. Not only the history of development but also the most recent advances are well covered and introduced, providing a highly meaningful reference for advancing the informatization and digitization of TCM. We hope this survey can well help both junior and experienced researchers in the relative areas.
Acknowledgement: The authors extend their appreciation to all who have contributed to the field of study, and to the anonymous reviewers for their insightful comments and suggestions that have significantly enhanced the quality of this paper.
Funding Statement: This work is supported by the research “Evidence Study Based on Multimodal Knowledge Graph Reasoning of the Idea of Treating Pre-disease in TCM (2023016)” and the National Natural Science Foundation of China (No. 82374621).
Author Contributions: The authors confirm contribution to the paper as follows: Study conception and design: Xiaolong Qu, Dongmei Li, Ziwei Tian; data collection: Xiaolong Qu, Dongmei Li, Jinman Cui, Ruowei Li; analysis and interpretation of results: Xiaolong Qu, Ziwei Tian, Ruowei Li; draft manuscript preparation: Xiaolong Qu, Xiaoping Zhang, Dongmei Li, Ziwei Tian. All authors reviewed the results and approved the final version of the manuscript.
Availability of Data and Materials: Not applicable.
Ethics Approval: Not applicable.
Conflicts of Interest: The authors declare no conflicts of interest to report regarding the present study.
References
1. G. Nestler, “Traditional Chinese medicine,” Med. Clin., vol. 86, no. 1, pp. 63–73, 2002. doi: 10.1016/S0025-7125(03)00072-5. [Google Scholar] [PubMed] [CrossRef]
2. J. Tang, B. Liu, and K. Ma, “Traditional Chinese medicine,” Lancet, vol. 372, no. 9654, pp. 1938–1940, 2008. doi: 10.1016/S0140-6736(08)61354-9. [Google Scholar] [PubMed] [CrossRef]
3. D. C. Tian et al., “A review of traditional Chinese medicine diagnosis using machine learning: Inspection, auscultation-olfaction, inquiry, and palpation,” Comput. Biol. Med., vol. 170, 2024, Art. no. 108074. doi: 10.1016/j.compbiomed.2024.108074. [Google Scholar] [PubMed] [CrossRef]
4. A. Hogan et al., “Knowledge graphs,” ACM Comput. Surv., vol. 54, pp. 1–37, 2021. [Google Scholar]
5. T. Yu et al., “Knowledge graph for TCM health preservation: Design, construction, and applications,” Artif. Intell. Med., vol. 77, no. 11, pp. 48–52, 2017. doi: 10.1016/j.artmed.2017.04.001. [Google Scholar] [PubMed] [CrossRef]
6. M. Hou, R. Wei, L. Lu, X. Lan, and H. Cai, “Research review of knowledge graph and its application in medical domain,” J. Comput. Res. Develop., vol. 55, pp. 2587–2599, 2018. [Google Scholar]
7. Z. Xu, Y. Sheng, L. He, and Y. Wang, “Review on knowledge graph techniques,” (in ChineseJ. Univ. Elect. Sci. Tech. China, vol. 45, no. 4, pp. 589–606, 2016. doi: 10.3969/j.issn.1001-0548.2016.04.012. [Google Scholar] [CrossRef]
8. S. Wang, Z. Li, T. Yang, and K. Hu, “Current status and development trend of knowledge graph research in traditional Chinese medicine,” (in ChineseJ. Nanjing Univ. Trad. Chin. Med., vol. 38, pp. 272–278, 2022. doi: 10.14148/j.issn.1672-0482.2022.0272. [Google Scholar] [CrossRef]
9. X. Shen et al., “Constructing the traditional Chinese medicine model of risk assessment for the recurrence of ischemic stroke: Based on multimodal network,” (in ChineseJ. Tradit. Chin. Med., vol. 63, pp. 725–729, 2022. doi: 10.13288/j.11-2166/r.2022.08.006. [Google Scholar] [CrossRef]
10. R. Li, Q. Xu, Y. Wu, and X. Liu, “Construction of multimodal knowledge graph based on materia medica,” Modern Comput., vol. 28, pp. 10–17, 2022. [Google Scholar]
11. H. Weng et al., “A framework for automated knowledge graph construction towards traditional Chinese medicine,” in Int. Conf. Health Inform. Sci., Moscow, Russia, 2017, pp. 170–181. [Google Scholar]
12. D. Zhai, Y. Lou, H. Kan, X. He, G. Liang and Z. Ma, “Constructing TCM knowledge graph with multi-source heterogeneous data,” Data Anal. Know. Disc., vol. 7, pp. 146–158, 2023. [Google Scholar]
13. Z. Liu, M. Sun, Y. Lin, and R. Xie, “Knowledge representation learning: A review,” J. Comput. Res. Dev., vol. 53, pp. 247–261, 2016. [Google Scholar]
14. T. Yu, “Knowledge engineering for traditional Chinese medicine: A review of theoretical system and key technologies,” Know. Manag. Forum, vol. 1, pp. 336–343, 2016. [Google Scholar]
15. L. Yuan, H. Zhang, J. Chen, and J. Lu, “Design pattern of knowledge base based on ontology knowledge model,” Comput. Eng. Appl., vol. 30, pp. 65–68, 2006. [Google Scholar]
16. C. Brewster and K. O'Hara, “Knowledge representation with ontologies: The present and future,” IEEE Intell. Syst., vol. 19, no. 1, pp. 72–81, 2004. doi: 10.1109/MIS.2004.1265889. [Google Scholar] [CrossRef]
17. W. Fund and O. Bodenreider, “Knowledge representation and ontologies,” in Clinical Research Informatics. Cham: Springer International Publishing, pp. 367–388, 2023. [Google Scholar]
18. D. Zhang, Y. Xie, M. Li, and C. Shi, “Construction of knowledge graph of traditional Chinese medicine based on the ontology,” Tech. Intell. Eng., vol. 3, pp. 35–42, 2017. [Google Scholar]
19. Z. Zeng et al., “Review on the research and application of knowledge graph and its key technologies in the field of traditional Chinese medicine,” (in ChineseModern. Trad. Chin. Med. Materia Medica-World Sci. Techol., vol. 24, no. 2, pp. 780–788, 2022. doi: 10.11842/wst.20210428006. [Google Scholar] [CrossRef]
20. H. Sun, H. Li, Y. Nie, and S. Zhen, “Research progress in knowledge graph and its application in the field of TCM,” (in ChineseModern. Trad. Chin. Med. Materia Medica-World Sci. Techol., vol. 22, no. 6, pp. 1969–1974, 2020. doi: 10.11842/wst.20190429007. [Google Scholar] [CrossRef]
21. S. Parreiras and S. Staab, “Using ontologies with UML class-based modeling: The twouse approach,” Data & Know. Eng., vol. 69, no. 11, pp. 1194–1207, 2010. doi: 10.1016/j.datak.2010.07.009. [Google Scholar] [CrossRef]
22. M. Abubakar, H. Hamdan, N. Mustapha, and T. Aris, “Instance-based ontology matching: A literature review,” in Int. Conf. Soft Comput. Data Min., Johor, Malaysia, 2018, pp. 455–469. [Google Scholar]
23. L. Zhu et al., “Construction of ontology of asthma in traditional Chinese medicine,” (in ChineseChin. J. Exper. Trad. Med. Form., vol. 23, no. 15, pp. 222–226, 2017. doi: 10.13422/j.cnki.syfjx.2017150222. [Google Scholar] [CrossRef]
24. Y. Cui, M. Wang, X. Chen, L. Zhang, and G. Li, “Construction for ontology of acne in traditional Chinese medicine,” (in ChineseModern. Trad. Chin. Med. Materia Medica-World Sci. Techol., vol. 21, no. 12, pp. 2867–2872, 2019. doi: 10.11842/wst.20181210002. [Google Scholar] [CrossRef]
25. Q. Liu, W. Zhong, and J. Yan, “Research on knowledge representation and reasoning methods of Chinese medicine,” (in ChineseJ. Basic Chin. Med., vol. 27, no. 9, pp. 1426–1429, 2021. [Google Scholar]
26. L. Tian, J. Zhang, J. Zhang, W. Zhou, and X. Zhou, “Knowledge graph survey: Representation, construction, reasoning and knowledge hypergraph theory,” J. Comput. Appl., vol. 41, pp. 2161–2186, 2021. [Google Scholar]
27. A. Bordes, N. Usunier, A. García-Durán, J. Weston, and O. Yakhnenko, “Translating embeddings for modeling multi-relational data,” in Adv. Neural Inform. Process. Syst., Lake Tahoe, CA, USA, Curran Associates, 2013, pp. 2787–2795. [Google Scholar]
28. Z. Wang, J. W. Zhang, J. L. Feng, and Z. Chen, “Knowledge graph embedding by translating on hyperplanes,” in Proc. AAAI Conf. Artif. Intell., Québec City, QC, Canada, 2014, pp. 1112–1119. [Google Scholar]
29. L. Ji, S. He, L. Xu, K. Liu, and J. Zhao, “Knowledge graph embedding via dynamic mapping matrix,” in Proc. 53rd Annual Meet. Assoc. Comput. Ling. 7th Int. Joint Conf. Natural Lang. Process., Beijing, China, 2015, pp. 687–696. [Google Scholar]
30. Y. Lin, Z. Liu, M. Sun, Y. Liu, and X. Zhu, “Learning entity and relation embeddings for knowledge graph completion,” in Proc. AAAI Conf. Artif. Intell., Austin, Texas, TX, USA, 2015, pp. 2181–2187. [Google Scholar]
31. Z. Li, Q. Zhang, F. Zhu, D. Li, C. Zheng and Y. Zhang, “Knowledge graph representation learning with simplifying hierarchical feature propagation,” Inform. Process. & Manag., vol. 60, no. 4, 2023, Art. no. 103348. doi: 10.1016/j.ipm.2023.103348. [Google Scholar] [CrossRef]
32. Z. Li et al., “Text-enhanced knowledge graph representation learning with local structure,” Inform. Process. Manag., vol. 61, no. 5, 2024, Art. no. 103797. doi: 10.1016/j.ipm.2024.103797. [Google Scholar] [CrossRef]
33. P. Bryan, A. Rami, and S. Steven, “DeepWalk: Online learning of social representations,” in Proc. 20th ACM SIGKDD Int. Conf. Know. Disc. Data Mining, New York, NY, USA, 2014. [Google Scholar]
34. G. Aditya and L. Jure, “Node2vec: Scalable feature learning for networks,” in Proc. 22nd ACM SIGKDD Int. Conf. Know. Disc. Data Min., San Francisco, CA, USA, 2016, pp. 855–864. [Google Scholar]
35. J. Tang, M. Qu, M. Wang, M. Zhang, J. Yan and Q. Mei, “LINE: Large-scale information network embedding,” in Proc. 24th Int. Conf. World Wide Web, Florence, Italy, 2015, pp. 1067–1077. [Google Scholar]
36. Z. Zheng, “Research on knowledge graph learning and reasoning for TCM prescription of chronic kidney disease,” Univ. of Electronic Science and Technology of China: China; 2020. [Google Scholar]
37. X. Wang, Y. Zhang, X. Wang, and J. Chen, “A knowledge graph enhanced topic modeling approach for herb recommendation,” in Int. Conf. Database Syst. Adv. Appl., Chiang Mai, Thailand, 2019, pp. 709–724. [Google Scholar]
38. Y. Jin, W. Zhang, X. He, X. Wang, and X. Wang, “Syndrome-aware herb recommendation with multi-graph convolution network,” in 2020 IEEE 36th Int. Conf. Data Eng. (ICDE), 2020, pp. 145–156. [Google Scholar]
39. Y. Yang, Y. Rao, M. Yu, and Y. Kang, “Multi-layer information fusion based on graph convolutional network for knowledge-driven herb recommendation,” Neural Netw., vol. 146, no. 6, pp. 1–10, 2022. doi: 10.1016/j.neunet.2021.11.010. [Google Scholar] [PubMed] [CrossRef]
40. C. Friedman, P. Alderson, J. Austin, J. Cimino, and S. Johnson, “A general natural-language text processor for clinical radiology,” J. Am. Med. Inform. Assoc., vol. 1, no. 2, pp. 161–174, 1994. doi: 10.1136/jamia.1994.95236146. [Google Scholar] [PubMed] [CrossRef]
41. Y. Wang, Z. Yu, Y. Jiang, Y. Liu, L. Chen and Y. Liu, “A framework and its empirical study of automatic diagnosis of traditional Chinese medicine utilizing raw free-text clinical records,” J. Biomed. Inform., vol. 45, no. 2, pp. 210–223, 2012. doi: 10.1016/j.jbi.2011.10.010. [Google Scholar] [PubMed] [CrossRef]
42. J. Gao et al., “Research on named entity extraction of TCM clinical medical records symptoms based on conditional random field,” (in ChineseModern. Trad. Chin. Med. Mat. Medica-World Sci. Technol., vol. 22, no. 6, pp. 1947–1954, 2020. [Google Scholar]
43. K. Liu, X. Zhou, J. Yu, and R. Zhang, “Named entity extraction of traditional Chinese medicine medical records based on conditional random field,” Comput. Eng., vol. 40, pp. 312–316, 2014. [Google Scholar]
44. S. Wang, S. Li, and T. Chen, “Recognition of Chinese medicine named entity based on condition random field,” (in ChineseJ. Xiamen Univ. (Nat. Sci.), vol. 48, no. 3, pp. 359–364, 2009. [Google Scholar]
45. W. Li, D. Zhao, B. Li, X. Peng, and J. Liu, “Combining CRF and rule based medical named entity recognition,” Appl. Res. Comput., vol. 32, pp. 1082–1086, 2015. [Google Scholar]
46. J. Liang et al., “A novel approach towards medical entity recognition in Chinese clinical text,” J. Healthc. Eng., vol. 1, no. 2, 2017, Art. no. 4898963. doi: 10.1155/2017/4898963. [Google Scholar] [PubMed] [CrossRef]
47. N. Yuan et al., “Depth representation-based named entity extraction for symptom phenotype of TCM medical record,” (in ChineseModern. Trad. Chin. Med. Mat. Medica-World Sci. Technol., vol. 20, no. 3, pp. 355–362, 2018. [Google Scholar]
48. N. Deng, H. Fu, and X. Chen, “Named entity recognition of traditional Chinese medicine patents based on BiLSTM-CRF,” Wirel. Commun. Mob. Comput., vol. 1, 2021, Art. no. 6696205. doi: 10.1155/2021/6696205. [Google Scholar] [CrossRef]
49. J. Gao, T. Yang, H. Dong, H. Shi, and K. Hu, “Study on named entity extraction of TCM clinical medical records symptoms based on LSTM-CRF,” (in ChineseChin. J. Inform. Trad. Chin. Med., vol. 28, no. 5, pp. 20–24, 2021. doi: 10.19879/j.cnki.1005-5304.202002142. [Google Scholar] [CrossRef]
50. G. Zheng, T. Yi, D. Tang, and S. He, “The Chinese ethnic medicine knowledge extraction based on BERT-BiLSTM-CRF model,” (in ChineseJ. Wuhan Univ. (Nat. Sci. Ed.), vol. 67, no. 5, pp. 393–402, 2021. doi: 10.14188/j.1671-8836.2020.0225. [Google Scholar] [CrossRef]
51. M. Zhang, Z. Yang, C. Liu, and L. Fang, “Traditional Chinese medicine knowledge service based on semi-supervised BERT-BiLSTM- CRF model,” in 2020 Int. Conf. Service Sci. (ICSS), Xining, China, 2020, pp. 64–69. [Google Scholar]
52. Q. Jia, D. Zhang, H. Xu, and Y. Xie, “Extraction of traditional Chinese medicine entity: Design of a novel span-level named entity recognition method with distant supervision,” JMIR Med. Inform., vol. 9, no. 6, 2021, Art. no. e28219. doi: 10.2196/28219. [Google Scholar] [PubMed] [CrossRef]
53. Z. Jin, Y. Zhang, H. Kuang, L. Yao, W. Zhang and Y. Pan, “Named entity recognition in traditional Chinese medicine clinical cases combining BiLSTM-CRF with knowledge graph,” in Int. Conf. Know. Sci., Eng. Manag., Athens, Greece, 2019, pp. 537–548. [Google Scholar]
54. M. Cheng, S. Xiong, F. Li, P. Liang, and J. Gao, “Multi-task learning for Chinese clinical named entity recognition with external knowledge,” BMC Med. Inform. Decis. Mak., vol. 21, no. 1, pp. 1–11, 2021. doi: 10.1186/s12911-021-01717-1. [Google Scholar] [PubMed] [CrossRef]
55. L. Duan, Q. Xu, A. Li, and M. Cui, “Research on effect of entities semantic information on Chinese entity relation extraction,” Appl. Res. Comput., vol. 34, pp. 141–146, 2017. [Google Scholar]
56. Y. Fang, H. Huang, H. Chen, and F. Juan, “TCMGeneDIT: A database for associated traditional Chinese medicine, gene and disease information using text mining,” BMC Complement. Altern. Med., vol. 8, no. 1, pp. 1–11, 2008. doi: 10.1186/1472-6882-8-58. [Google Scholar] [PubMed] [CrossRef]
57. T. Yu, L. Zhu, J. Li, and H. Gao, “Text information extraction system for traditional Chinese medicine domain,” (in ChineseMed. Innov. China, vol. 12, no. 21, pp. 108–110, 2015. doi: 10.3969/j.issn.1674-4985.2015.21.040. [Google Scholar] [CrossRef]
58. X. Yang, Y. Shan, D. Xie, and X. Li, “Relation extraction of traditional Chinese medicine prescription and disease based on literature abstracts data,” (in ChineseModern. Trad. Chin. Med. Materia Medica-World Sci. Technol., vol. 19, no. 7, pp. 1167–1172, 2017. [Google Scholar]
59. J. Luo, J. Du, B. Nie, W. Xiong, L. Liu and J. He, “TCM text relation extraction model based on bidirectional LSTM and GBDT,” Appl. Res. Comput., vol. 36, pp. 3744–3747, 2019. [Google Scholar]
60. H. Wan et al., “Extracting relations from traditional Chinese medicine literature via heterogeneous entity networks,” J. Am. Med. Inform. Assoc., vol. 23, no. 2, pp. 356–365, 2016. doi: 10.1093/jamia/ocv092. [Google Scholar] [PubMed] [CrossRef]
61. T. Bai, H. Guan, S. Wang, Y. Wang, and L. Huang, “Traditional Chinese medicine entity relation extraction based on CNN with segment attention,” Neural Comput. Appl., vol. 34, no. 4, pp. 2739–2748, 2022. doi: 10.1007/s00521-021-05897-9. [Google Scholar] [CrossRef]
62. X. Han, X. Li, Y. Liang, X. Wang, D. Xu and R. Guan, “Acupuncture and Tuina knowledge graph for ancient literature of traditional Chinese medicine,” in 2021 IEEE Int. Conf. Bioinform. Biomed. (BIBM), Houston, TX, USA, 2021, pp. 674–677. [Google Scholar]
63. H. Lin, Y. Wang, Y. Jia, P. Zhang, and W. Wang, “Network big data oriented knowledge fusion methods: A survey,” (in ChineseChin. J. Comput., vol. 40, no. 1, pp. 1–27, 2017. [Google Scholar]
64. W. Hao, J. Yu, and X. Zhou, “The overview of ontology alignment techniques and their applications to traditional Chinese medicine (TCM),” Modern. Trad. Chin. Med. Materia Medica-World Sci. Technol., vol. 19, pp. 63–69, 2017. [Google Scholar]
65. J. Zhang, X. Zhang, C. Wu, and Z. Zhao, “Survey of knowledge graph construction techniques,” Comput. Eng., vol. 48, pp. 23–37, 2022. [Google Scholar]
66. F. Miao, H. Liu, Y. Huang, C. Liu, and X. Wu, “Construction of semantic-based traditional Chinese medicine prescription knowledge graph,” in 2018 IEEE 3rd Adv. Inform. Technol., Elect. Autom. Cont. Conf. (IAEAC), Chongqing, China, 2018, pp. 1194–1198. [Google Scholar]
67. W. Guo, “Research and implementation of knowledge mapping of traditional Chinese medicine prescription,” Ph.D. thesis, Lanzhou Univ., Lanzhou, China, 2019. [Google Scholar]
68. Y. Zhang, “Research and construction of tongue image diagnosis and treatment system based on knowledge graph,” Ph.D. thesis, Univ. of Electronic Science and Technology of China, Chengdu, China, 2019. [Google Scholar]
69. Q. Lou, S. Wang, J. Chen, D. Mu, Y. Wang and L. Huang, “Named entity recognition of traditional Chinese medicine cases based on RoBERTa-BiLSTM-CRF,” in 2023 IEEE Int. Conf. Bioinform. Biomed. (BIBM), Istanbul, Turkey, 2023, pp. 4609–4614. [Google Scholar]
70. Y. Zhang, “Construction of knowledge map on experience in TCM prescriptions of dermatology schools of zhao bingnan and zhu renkang,” (in ChineseChin. J. Library and Inform., vol. 45, no. 2, pp. 1–5, 2021. doi: 10.3969/j.issn.2095-5707.2021.02.001. [Google Scholar] [CrossRef]
71. K. Zhang, X. Li, Y. Yan, Q. Zhu, and J. Ma, “Domain expert entity extraction method based on multi-feature bidirectional gated neural network,” J. Nanjing Normal Univ. (Natur. Sci. Ed.), vol. 44, pp. 128–135, 2021. [Google Scholar]
72. S. Yang, X. H. Wang, Z. G. Zhao, J. S. Pan, and L. Wu, “Research on the construction and application of COVID-19 knowledge graph,” J. Qingdao Univ. (Eng. Technol. Ed.), vol. 36, no. 4, pp. 22–29, 2021. doi: 10.13306/j.1006-9798.2021.04.004. [Google Scholar] [CrossRef]
73. W. Dong, S. Sun, and M. Yin, “Research and development of medical knowledge graph reasoning,” J. Front. Comput. Sci. Technol., vol. 16, pp. 1193–1213, 2022. [Google Scholar]
74. X. Chen, S. Jia, and Y. Xiang, “A review: Knowledge reasoning over knowledge graph,” Expert. Syst. Appl., vol. 141, no. 6, 2020, Art. no. 112948. doi: 10.1016/j.eswa.2019.112948. [Google Scholar] [CrossRef]
75. Y. Xie, L. Jia, and J. Dai, “Construction of a traditional Chinese medicine dao yin science knowledge graph based on Neo4j*,” in 2023 IEEE Int. Conf. Bioinform. Biomed. (BIBM), Istanbul, Turkey, 2023, pp. 4662–4666. [Google Scholar]
76. X. Wang, T. Yang, X. Gao, and K. Hu, “Knowledge graph enhanced transformers for diagnosis generation of Chinese medicine,” Chin. J. Integr. Med., vol. 30, pp. 267–276, 2024. doi: 10.1007/s11655-023-3612-5. [Google Scholar] [PubMed] [CrossRef]
77. H. Long et al., “Towards an ontology-based decision support system for syndrome-differentiation and treatment of Psoriasis Vulgaris,” Trad. Chin. Med., 2020. doi: 10.21203/rs.3.rs-38383/v1. [Google Scholar] [CrossRef]
78. C. Yang, W. Li, X. Zhang, R. Zhang, and G. Qi, “A temporal semantic search system for traditional Chinese medicine based on temporal knowledge graphs,” in Joint Int. Semantic Technol. Conf., Hangzhou, China, 2019, pp. 13–20. [Google Scholar]
79. Z. Fu et al., “Diagnosis and treatment reasoning of integrated traditional Chinese and western medicine against acute abdomen based on knowledge graph,” (in ChineseChin. J. Experim. Trad. Medical Form., vol. 29, no. 11, pp. 190–199, 2023. doi: 10.13422/j.cnki.syfjx.20230512. [Google Scholar] [CrossRef]
80. Y. Bao, “Research on construction of knowledge base and knowledge discovery of traditional Mongolian medicine based on domain ontology,” Ph.D. thesis, Jilin Univ., Changchun, China, 2018. [Google Scholar]
81. Y. Xie, L. Hu, X. Chen, J. Feng, and D. Zhang, “Auxiliary diagnosis based on the knowledge graph of tcm syndrome,” Comput. Mater. Contin., vol. 65, pp. 481–494, 2020. doi: 10.32604/cmc.2020.010297. [Google Scholar] [CrossRef]
82. F. Yang et al., “Research on knowledge representation, acquisition and discovery of ancient Chinese medicine books based on knowledge element indexing and knowledge graph,” J. Basic Chin. Med., vol. 29, no. 6, pp. 954–959, 2023. [Google Scholar]
83. J. Wang, L. Xiao, and J. Yan, “Research on construction of knowledge graph of treatise on febrile diseases based on Neo4j,” (in ChineseComput. & Digital Eng., vol. 49, no. 2, pp. 264–267, 2021. doi: 10.3969/j.issn.1672-9722.2021.02.007. [Google Scholar] [CrossRef]
84. M. Jia, J. Li, J. Liu, Y. Yin, Y. Wang and L. Xu, “Study on application of knowledge graph in TCM pediatric treatment of Disease,” in 2021 IEEE Int. Conf. Bioinform. Biomed. (BIBM), Houston, TX, USA, 2021, pp. 3818–3821. [Google Scholar]
85. R. Zuo et al., “Research on the application of knowledge graph in the academic experience inheritance of distinguished TCM veteran doctors,” (in ChineseChina Digit. Med., vol. 16, pp. 33–36, 2021. [Google Scholar]
86. F. Yang et al., “Knowledge representation, acquisition and retrieval of ancient traditional Chinese medicine books based on knowledge element-semantic network model,” 2022. doi: 10.2139/ssrn.4068444. [Google Scholar] [CrossRef]
87. Y. Xie, C. Yan, and D. Zhang, “Personalized diagnostic modal discovery of traditional Chinese medicine knowledge graph,” in 2018 14th Int. Conf. Natural Comput., Fuzzy Syst. Know. Disc. (ICNC-FSKD), Huangshan, China, 2018, pp. 1096–1103. [Google Scholar]
88. K. Zhao, H. Wang, N. Shi, Z. Sha, and X. Xu, “Study and implementation on knowledge graph of guizhi decoction associated formulas based on Neo4j,” (in ChineseWorld Chin. Med., vol. 14, no. 10, pp. 2636–2639, 2019. doi: 10.3969/j.issn.1673-7202.2019.10.019. [Google Scholar] [CrossRef]
89. D. Yin, L. Zhou, Y. Zhou, Y. Sun, and Y. Li, “Study on design of graph search pattern of knowledge graph of TCM classic prescriptions,” (in ChineseChin. J. Inform. Trad. Chin. Med., vol. 26, no. 8, pp. 94–98, 2019. doi: 10.3969/j.issn.1005-5304.2019.08.019. [Google Scholar] [CrossRef]
90. L. Liu and X. Li, “Research and construction of classical formulas knowledge graph based on ontology,” in WI-IAT'21: IEEE/WIC/ACM Int. Conf. Web Intell. Intellig. Agent Technol., Melbourne, Australia, 2021, pp. 140–143. [Google Scholar]
91. L. Liu and X. Li, “Research and construction of marine Chinese medicine formulas knowledge graph,” in 2021 IEEE Int. Conf. Bioinform. Biomed. (BIBM), Houston, TX, USA, 2021, pp. 3853–3855. [Google Scholar]
92. Y. Chen et al., “Design of knowledge graph of traditional Chinese medicine prescription and knowledge analysis of implicit relation,” in ISAIMS'20: Proc. 1st Int. Symp. Artif. Intell. Med. Sci., Beijing, China, 2020, pp. 56–63. [Google Scholar]
93. R. Xiao, F. Hu, W. Pei, and M. Bie, “Research on traditional Chinese medicine data mining model based on traditional Chinese medicine basic theories and knowledge graphs,” in ISAIMS'20: Proc. 1st Int. Symp. Artif. Intell. Med. Sci., Beijing, China, 2020, pp. 102–106. [Google Scholar]
94. H. Hong, S. Wang, W. Li, and L. Yang, “Research on data mining and knowledge completion based on Chinese marine medicine knowledge graph,” Inform. TCM, vol. 40, pp. 27–34, 2023. [Google Scholar]
95. M. Xiao, “Research on construction and application of knowledge graph of health domain for traditional Chinese medicine syndrome,” M.S. thesis, Jilin Univ., Changchun, China, 2019. [Google Scholar]
96. B. Ye, Y. Zhou, and Y. Le, “Study on the knowledge map of pathogenesis of chest paralysis based on ancient books of Chinese medicine,” Asia-Pacific Trad. Med., vol. 16, pp. 153–155, 2020. [Google Scholar]
97. T. Ruan, C. Sun, H. Wang, Z. Fang, and Y. Yin, “Construction of traditional Chinese medicine knowledge graph and its application,” J. Med. Intell., vol. 37, pp. 8–13, 2016. [Google Scholar]
98. B. Cheng, Y. Zhang, D. Cai, W. Qiu, and D. Shi, “Construction of traditional Chinese medicine knowledge graph using data mining and expert knowledge,” in 2018 Int. Conf. Netw. Infrastruct. Digital Content (IC-NIDC), Guiyang, China, 2018, pp. 209–213. [Google Scholar]
99. Z. Liu, E. Peng, S. Yan, G. Li, and T. Hao, “T-know: A knowledge graph-based question answering and information retrieval system for traditional Chinese medicine,” in Proc. 27th Int. Conf. Comput. Linguistics: System Demonst., Santa Fe, NM, USA, 2018, pp. 15–19. [Google Scholar]
100. Y. Lv, “Construction of knowledge graph oriented to questions and answers of traditional Chinese medicine and research on problem representation algorithm,” Ph.D. thesis, Beijing Jiaotong Univ., Beijing, China, 2020. [Google Scholar]
101. M. N. Du, C. J. Hu, F. Ye, L. Jin, and K. F. Hu, “Research on construction of knowledge graph and link prediction for diagnosis and treatment of brain tumors by TCM master Zhou Zhongying,” Softw. Guide., vol. 22, no. 7, pp. 1–7, 2023. doi: 10.11907/rjdk.231216. [Google Scholar] [CrossRef]
102. Y. Yin, G. Li, Y. Wang, Q. Zhang, M. Wang and L. Zhang, “Study on construction and application of knowledge graph of TCM diagnosis and treatment of viral hepatitis B,” in 2021 IEEE Int. Conf. Bioinform. Biomed. (BIBM), Houston, TX, USA, 2021, pp. 3906–3911. [Google Scholar]
103. M. Sun, D. Zhang, M. Zheng, and S. Mei, “Traditional Chinese medicine aided diagnosis and treatment system for rheumatoid arthritis based on artificial intelligence,” Pattern Recognit Artif. Intell., vol. 34, pp. 343–352, 2021. [Google Scholar]
104. Y. Deng, W. Zhou, Z. Zhang, Z. Ning, and Y. Tan, “Construction of knowledge map based on famous old Chinese medicine case,” (in ChineseHunan J. Trad. Chin. Med., vol. 35, no. 7, pp. 186–187, 2019. [Google Scholar]
105. L. Jia, L. Liu, J. Liu, and B. Gao, “Design and construction of knowledge-based question answering system based on the language system for traditional Chinese medicine,” (in ChineseChin. J. Med. Libr. Inform. Sci., vol. 28, no. 5, pp. 11–14, 2019. doi: 10.3969/j.issn.1671-3982.2019.05.003. [Google Scholar] [CrossRef]
106. Y. Gao et al., “Research on the knowledge graph construction of auxiliary notes on drugs and methods for internal organs diseases based on graph database,” (in ChineseChina Digit. Med., vol. 18, pp. 92–98, 2023. [Google Scholar]
107. Z. Zheng, Y. Liu, Y. Zhang, and G. Wen, “TCMKG: A deep learning based traditional Chinese medicine knowledge graph platform,” in 2020 IEEE Int. Conf. Know. Graph (ICKG), Nanjing, China, 2020, pp. 560–564. [Google Scholar]
108. T. Ruan et al., “An automatic approach for constructing a knowledge base of symptoms in Chinese,” in 2016 IEEE Int. Conf. Bioinform. Biomed. (BIBM), Shenzhen, China, 2016, pp. 1657–1662. [Google Scholar]
109. Y. Zhong, C. Ru, B. Zhang, and Y. Cheng, “Studies on the methodology for quality control in Chinese medicine manufacturing process based on knowledge graph,” (in ChineseChina J. Chin. Mater. Med., vol. 44, pp. 5269–5276, 2019. doi: 10.19540/j.cnki.cjcmm.20191203.302. [Google Scholar] [PubMed] [CrossRef]
110. Y. Deng, H. Huang, Z. Zhang, W. Zhou, and Y. Gong, “Research on the construction of the knowledge sharing platform for inheritance and innovation of traditional Chinese medicine,” (in ChineseChina Digit. Med., vol. 15, pp. 66–69, 2020. [Google Scholar]
111. R. Liu, L. Zhu, X. Sun, and Y. Sun, “Construction for ontology of stroke in traditional Chinese medicine,” in 2023 IEEE Int. Conf. Bioinform. Biomed. (BIBM), Istanbul, Turkey, 2023, pp. 4603–4608. [Google Scholar]
112. R. Yang, Q. Ye, C. Cheng, S. Zhang, Y. Lan and J. Zou, “Decision-making system for the diagnosis of syndrome based on traditional Chinese medicine knowledge graph,” Evid. Based Complement. Alternat. Med., vol. 2022, pp. 1–9, 2022. doi: 10.1155/2022/8693937. [Google Scholar] [PubMed] [CrossRef]
113. D. Li, J. Qu, Z. Tian, Z. Mou, L. Zhang and X. Zhang, “Knowledge-based recurrent neural network for TCM cerebral palsy diagnosis,” Evid. Based Complement Alternat. Med., vol. 2022, no. 1, pp. 1–10, 2022. doi: 10.1155/2022/7708376. [Google Scholar] [PubMed] [CrossRef]
114. S. Wen, “Design and implementation of automatic question answering system based on ontology for traditional Chinese medicine coronary artery disease,” Ph.D. thesis, Shenyang Univ. of Technology, Shenyang, China, 2017. [Google Scholar]
115. Y. Yin, L. Zhang, Y. Wang, M. Wang, Q. Zhang and G. Li, “Question answering system based on knowledge graph in traditional Chinese medicine diagnosis and treatment of viral hepatitis B,” Biomed Res. Int., vol. 2022, no. 2, pp. 1–8, 2022. doi: 10.1155/2022/7139904. [Google Scholar] [PubMed] [CrossRef]
116. R. Gao and C. Li, “Knowledge question-answering system based on knowledge graph of traditional Chinese medicine,” in 2020 IEEE 9th Joint Int. Inform. Technol. Artif. Intell. Conf. (ITAIC), Chongqing, China, 2020, pp. 27–31. [Google Scholar]
117. Y. Zou, Y. He, and Y. Liu, “Research and implementation of intelligent question answering system based on knowledge Graph of traditional Chinese medicine,” in 2020 39th Chinese Control Conf. (CCC), Shenyang, China, 2020, pp. 4266–4272. [Google Scholar]
118. J. Luo, “Research and application of key technologies for knowledge graph in traditional Chinese medicine,” Ph.D. thesis, Jiangxi Univ. of Chinese Medicine, Nanchang, China, 2019. [Google Scholar]
119. T. Zhou, T. Yang, and K. Hu, “Traditional Chinese medicine epidemic prevention and treatment question-answering model based on LLMs,” in 2023 IEEE Int. Conf. Bioinform. Biomed. (BIBM), Istanbul, Turkey, 2023, pp. 4755–4760. [Google Scholar]
120. W. Zhao et al., “TCM herbal prescription recommendation model based on multi-graph convolutional network,” J. Ethnopharmacol., vol. 297, no. 3, 2022, Art. no. 115109. doi: 10.1016/j.jep.2022.115109. [Google Scholar] [PubMed] [CrossRef]
121. J. Qi, X. Wang, and T. Yang, “Traditional Chinese medicine prescription recommendation model based on large language models and graph neural networks,” in 2023 IEEE Int. Conf. Bioinform. Biomed. (BIBM), Istanbul, Turkey, 2023, pp. 4623–4627. [Google Scholar]
122. X. Qu et al., “TCM intelligent recommendation based on multimodal knowledge graph attention network,” in ICCIP'23: Proc. 2023 9th Int. Conf. Commun. Inform. Process., Hainan, China, 2023, pp. 631–637. [Google Scholar]
123. Z. Gong, N. Zhang, and J. He, “KGRN: Knowledge graph relational path network for target prediction of TCM prescriptions,” in Int. Conf. Intell. Comput., Shenzhen, China, 2021, pp. 148–161. [Google Scholar]
124. Z. Yin, J. Luo, Y. Tan, and Y. Zhang, “TCMCoRep: Traditional Chinese medicine data mining with contrastive graph representation learning,” in Int. Conf. Know. Sci., Eng. Manag., Guangzhou, China, 2023, pp. 44–55. [Google Scholar]
125. L. Huang et al., “TCMSSD: A comprehensive database focused on syndrome standardization,” Phytomedicine, vol. 128, 2024, Art. no. 155486. doi: 10.1016/j.phymed.2024.155486. [Google Scholar] [PubMed] [CrossRef]
126. C. Khoo, M. Baker, F. Zakaria, J. Chen, S. Ang and B. Huang, “Development of the polyglot asian medicine knowledge graph system,” in Int. Conf. Asian Digit. Libraries, Singapore: Springer Nature Singapore, 2023, pp. 3–11. [Google Scholar]
127. Z. Liu et al., “TCM-KDIF: An information interaction framework driven by knowledge-data and its clinical application in traditional Chinese medicine,” IEEE Internet Things J., vol. 11, no. 11, pp. 20002–20014, 2024. doi: 10.1109/JIOT.2024.3368029. [Google Scholar] [CrossRef]
128. Y. Qian et al., “Model informed precision medicine of Chinese herbal medicines formulas-a multi-scale mechanistic intelligent model,” J. Pharm. Anal., vol. 14, no. 4, 2024, Art. no. 100914. doi: 10.1016/j.jpha.2023.12.004. [Google Scholar] [PubMed] [CrossRef]
129. M. Zou, X. Huang, Y. Cheng, and X. Ning, “CNKI-based analysis of network pharmacology knowledge map in TCM,” (in ChineseWestern J. Trad. Chin. Med., vol. 37, no. 2, pp. 59–64, 2024. [Google Scholar]
Cite This Article
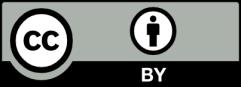
This work is licensed under a Creative Commons Attribution 4.0 International License , which permits unrestricted use, distribution, and reproduction in any medium, provided the original work is properly cited.