Open Access
ARTICLE
A Real-Time Semantic Segmentation Method Based on Transformer for Autonomous Driving
1 Donald Bren School of Information and Computer Sciences, University of California, Irvine, CA 92612, USA
2 Department of Control Engineering, Kyushu Institute of Technology, Kitakyushu, 804-8550, Japan
3 School of Automation, Southeast University, Nanjing, 210096, China
* Corresponding Author: Huimin Lu. Email:
(This article belongs to the Special Issue: Recognition Tasks with Transformers)
Computers, Materials & Continua 2024, 81(3), 4419-4433. https://doi.org/10.32604/cmc.2024.055478
Received 28 June 2024; Accepted 14 November 2024; Issue published 19 December 2024
Abstract
While traditional Convolutional Neural Network (CNN)-based semantic segmentation methods have proven effective, they often encounter significant computational challenges due to the requirement for dense pixel-level predictions, which complicates real-time implementation. To address this, we introduce an advanced real-time semantic segmentation strategy specifically designed for autonomous driving, utilizing the capabilities of Visual Transformers. By leveraging the self-attention mechanism inherent in Visual Transformers, our method enhances global contextual awareness, refining the representation of each pixel in relation to the overall scene. This enhancement is critical for quickly and accurately interpreting the complex elements within driving scenarios—a fundamental need for autonomous vehicles. Our experiments conducted on the DriveSeg autonomous driving dataset indicate that our model surpasses traditional segmentation methods, achieving a significant 4.5% improvement in Mean Intersection over Union (mIoU) while maintaining real-time responsiveness. This paper not only underscores the potential for optimized semantic segmentation but also establishes a promising direction for real-time processing in autonomous navigation systems. Future work will focus on integrating this technique with other perception modules in autonomous driving to further improve the robustness and efficiency of self-driving perception frameworks, thereby opening new pathways for research and practical applications in scenarios requiring rapid and precise decision-making capabilities. Further experimentation and adaptation of this model could lead to broader implications for the fields of machine learning and computer vision, particularly in enhancing the interaction between automated systems and their dynamic environments.Keywords
Cite This Article
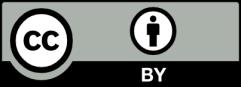
This work is licensed under a Creative Commons Attribution 4.0 International License , which permits unrestricted use, distribution, and reproduction in any medium, provided the original work is properly cited.